Just as crude oil fueled the industrial revolution, data drives the engines of the current digital age. This flood of data, underpinned by rapid advancement in AI and data analytics, fundamentally reshapes the finance functions inside organizations.
It's no longer a back-office number-cruncher; finance has evolved to become a strategic powerhouse for growth, performance optimization, and risk mitigation through the intelligent use of data. However, such transformation needs a strategic roadmap, along with deep knowledge of both technological capabilities and intrinsic peculiarities of financial operations.
Finance transformation has followed the broader technological development in data management and analytics. Initially, Enterprise Resource Planning (ERP) systems like SAP and Oracle integrated finance by dispersing processes, bringing together a centralized repository.
This paved the way for data warehouse-driven Business Intelligence (BI) dashboards for fast insights into historical trends and performance measures. Today, the emergence of data lakehouses further supports those capabilities of AI and data analytics that have come to mean a new frontier of predictive and prescriptive capabilities, thus setting finance functions on a path not just to understand the "what" and "why" of past performance, but also to anticipate the "what's next" and proactively shape their organizations' futures.
Finance leaders have always been adept at navigating complex financial landscapes. However, “know-how” isn't enough; we need to “know now.”
In other words, AI and data analytics are no longer optional extracts; they're valuable assets for discovering real-time insights, proactive decision-making, and predictive capabilities.
I've led large-scale transformations for major Financial Services institutions and enterprises and this experience has allowed me to witness the real issues that legacy systems cause in terms of agility and hindering strategic decisions.
What organizations need today is a finance team acting as strategic advisors - a group of professionals who can provide insight and foresight in real time to deal with emerging complexities and capitalize on opportunities. This involves a transformation brought about by four key objectives:
1. From transactional to strategic
Finance must shift from a transactional role, which is focused on recording and reporting, toward a proactive partner that contributes to business strategy and value creation. This represents a more basic change in mindset for finance professionals, using data and AI to identify trends, forecast outcomes, and drive strategic investments.
2. Operational excellence
Operational excellence is changing finance functions, not only by bringing down costs but also by releasing new levels of productivity. Robotic Process Automation (RPA) solutions automate much of the manual effort and human error involved in such processes as invoice processing and reconciliation, freeing resources for more strategic programs.
3. Regulatory landscape
Finance functions operate in an increasingly complex regulatory environment. Maintaining compliance with evolving standards like IFRS and GAAP ( or whichever regional standard may be at play, such as Chinese Accounting Standards (CAS) or Indian Accounting Standards (Ind AS) requires truly robust systems and processes to contain risk.
AI provides a robust solution through the automation of compliance checks, and flagging of potential violations in real-time, including but not limited to lease accounting errors under IFRS 16, and ensures regulatory reporting requirements are met related to generating XBRL reports for filing at the SEC. It would minimize any penalties and reputational losses by making the approach proactive and ensuring the accuracy and transparency of financial reporting.
4. Proactive risk management
Proactive identification and mitigation of financial risks are critical in attaining organizational resilience and ensuring sustainable growth. AI-powered systems can perform continuous monitoring of transactions to identify anomalies and send an early warning on potential fraud or financial misstatement with a timely warning for taking action on time to minimize the potential loss.
Artificial intelligence and data Analytics
AI and data analytics are no longer concepts of the future, but very real and important tools for today's finance functions. These are the ways whereby the finance function can pursue those above-mentioned objectives.
With a bundle of power from AI and data analytics, finance can take a quantum leap from being a reactive cost center to a proactive strategic partner.
Automation with RPA
RPA is one example of how finance departments have traditionally been doing repetitive rules-based activities. Right from invoice processing to data entry, reconciliation, and many others, companies can reduce manual efforts and errors by up to 50% if RPA is deployed. This would not only release valuable human capital for more strategic tasks in analysis and decision-making, but would also provide more accurate and effective results.
Predictive power of machine learning
ML algorithms are also allowing finance functions to move from historical reporting to predictive capabilities. The ML model can also forecast future trends by analyzing historical data and recognizing patterns, thus enabling appropriate budgeting, financial planning, and resource allocation. This, in turn, will allow organizations to predict any market changes, optimize resource utilization, and proactively make decisions for growth in profitability.
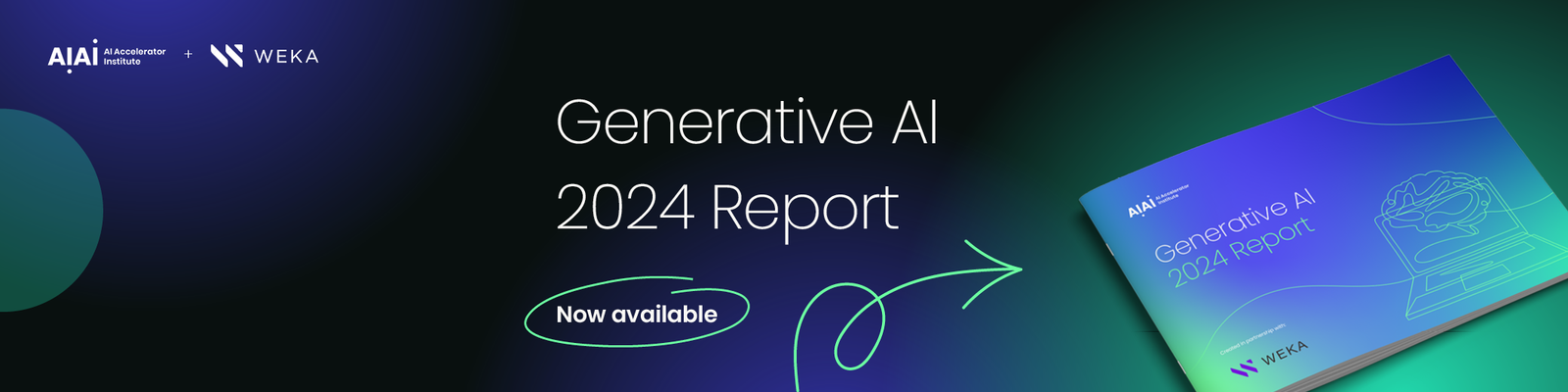
Unstructured data unlocks insights
NLP and LLMs now empower finance professionals to distill valuable insights from unstructured data sources like contracts, regulatory filings, reports, and news articles. This critical context helps drive decisions and allows the finance teams to deeply understand market dynamics, customer sentiment, and emerging risks. This will eventually enable organizations to make smarter decisions identify opportunities in advance and reduce potential threats proactively.
Data-driven decision making
Advanced analytics dashboards and visualizations are revolutionizing the way finance departments present and consume information. These tools provide instant insights into financial performance and drive data-based decisions throughout all levels of organizations. More importantly, it arms business leaders with exactly what they need to make the right decisions in a minimum amount of time and, therefore, faster to cope with the agile world.
Strengthen risk management
AI-powered systems go hand in hand with underpinning risk management in finance functions. These systems further grant compliance, avoid financial risks, and monitor on an ongoing basis transactions against anomalies and possible fraudulent activities. It is a proactive way of managing risks to help organizations protect their assets, maintain their reputation, and ensure long-term sustainability.
Key use cases: Where AI is making a real impact
The use of AI and data analytics in finance is not some vague concept; it's a reality being implemented by leading financial institutions to solve real-world challenges and drive tangible business value.
Here are some key use cases where AI is making a great impact in 2024, supported by recent reports and statistics:
Invoice processing
AI will revolutionize invoicing processes by automating tasks related to data extraction, invoice matching, and fraud detection. Key information from invoices can be automatically extracted independently of their form, either paper or digital, using AI-driven invoice processing solutions to prevent manual entry and reduce errors.
For example, the AI-driven document processing platform Rossum boasts it can achieve up to 98% accuracy in invoice data extraction, hence effectively boosting efficiency and increasing efficiency related to the processing time. Moreover, AI algorithms can execute the three-way matching of invoices with POs and receipts.
Account receivable
AI is also rebalancing accounts receivable by greatly improving credit scoring, collection, and cash flow forecasting. AI algorithms can analyze huge volumes of data on customer payment history, credit scores, and market trends to predict late payments and indicate customers who are most likely to present risk. Thus, AI enables business owners to take a proactive approach toward credit risk management and optimizing collections strategies.
Accounts payable operations
AI is smoothing the workflow of accounts payable by automating invoice processing, vendor management, and fraud prevention. As mentioned earlier, AI can do automatic data extraction in invoices and their matching, hence saving manual effort and reducing inaccuracies.
Also, AI can perform vendor data analysis to spot potential risks, such as financial instability or compliance issues, for proactive vendor management. Some AI algorithms can also identify various anomalies in invoice data, such as duplicate invoices or suspicious payment requests, to prevent fraudulent activities and compliance with internal controls.
Accounts reconciliation
The use of AI will surely continue to drive efficiency and effectiveness in account reconciliations with automated data matching, identification of discrepancies, and preparation of reconciliation reports. AI algorithms can sift through large volumes of transaction data, identify and clear matching exceptions, and update current statuses of reconciliations. This development reduces manual efforts and, therefore, cuts down on errors, thereby speeding up the process of reconciliation.
Predictive financial forecasting
AI models enhance financial forecasting by analyzing vast amounts of historical data, market trends, and external factors to generate more accurate predictions of revenue, expenses, and cash flow. This allows organizations to anticipate future financial performance, identify potential challenges, and make proactive adjustments to their strategies.
For example, Shell and BP have started implementing machine learning since 2017 to predict changes in energy markets for improved revenue predictions from global trends in energy consumption and pricing.
Automated financial reporting
RPA and AI are automating the generation of financial statements and reports, freeing up finance professionals from tedious manual tasks and reducing the risk of errors. This not only saves time and resources but also ensures greater accuracy and consistency in financial reporting.
For example, in a recent study, Gartner finds that 58% of organizations are using AI for financial reporting in everything from automated data extraction and reconciliation to variance analysis and anomaly detection. Large language models are being considered to parse legislation and regulations in countries where they operate to ensure each regulation is followed.
Fraud detection and compliance
AI algorithms are playing a crucial role in combating financial crime by monitoring transactions in real time, identifying suspicious patterns, and flagging potential fraud. This helps organizations stay ahead of increasingly sophisticated fraudsters and protect their financial assets.
A report by the Communications Fraud Control Association (CFCA) found that telecommunications fraud in the global telecom industry increased 12% in 2023 equating to an estimated $38.95 billion lost to fraud. AI-powered solutions can help analyze call records for anomaly detection and flag suspicious activities to help the operators reduce such losses and save their revenue streams.
Expense management automation
AI is streamlining expense management by automating expense reporting and reimbursement processes. By extracting data from purchase histories, and receipts, categorizing expenses, and ensuring compliance with company policies, AI reduces errors, saves time, and frees up employees from tedious administrative tasks.
For instance, retail companies like Walmart are into the use of AI mechanisms for automating purchase-ordering and expense management. Companies use AI-driven systems that study customer purchasing habits of the past and predict future needs to optimize products. This will help smoothen their procurement cycles and enhance overall operational effectiveness, saving a lot of manual efforts and reducing procurement costs.
Liquidity and cash management
Predictive analytics is optimizing cash flow forecasting, working capital management, and investment decisions, improving liquidity and financial stability. This allows organizations to better manage their cash flow, optimize working capital, and make informed investment decisions.
For example, big corporations like Hunt Companies have adopted the AI-powered Kyriba platform in their approach to real-time liquidity management. Equipped with integrated APIs, this platform makes working in the treasury easier by providing real-time visibility of cash flows for better capital allocation.
AI assists in liquidity management in all ways a firm might need because it further empowers the ability of a firm to handle the cash reserve with the help of predictive analytics, which can predict and deliver future liquidity needs, especially in times of turmoil in the markets.
In 2023, industries like healthcare also benefited from AI in managing treasury. Health Care Service Corporation employed AI-driven treasury data analytics and reinvented cash flow management to make better-working capital decisions. The move freed up over 1,000 hours of productivity by automating what had been manually-intensive cash management practices. With the power of AI-driven models, the company attained faster and more data-driven decisions that allowed it to manage liquidity through unpredictable financial cycles better.
Implementing AI in Finance
The transformation of the finance function with AI is not a plug-and-play exercise; rather, it calls for a structured approach, commitment toward change, and deep insight into both the technology and nuances of financial operations.
Below is a high-level roadmap highlighting the major phases a company undergoes during its AI finance implementation.
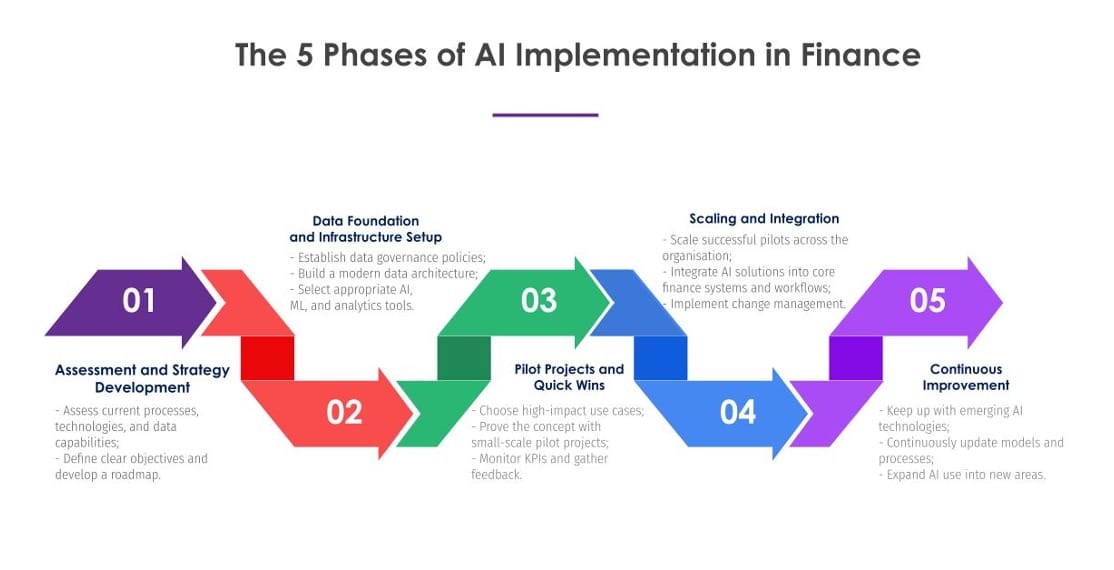
This phase-based approach to AI implementation in finance underlines the strategic process and iterative evolution, as opposed to a typical finance transformation that would center around ERP systems, data warehouses, and business intelligence dashboards.
While those technologies centered around centralization of data and reporting, this roadmap counts on data as a foundation for AI, with much stress on data governance, advanced analytics, and continuous improvement. It does this by pointing out that AI is a constantly evolving entity, and to keep up, agile adaptation is required, whereas most implementations of traditional systems are more rigid.
Besides that, it covers change management and cross-functional collaboration to ensure seamless integration of AI into the finance function and greater organzational objectives. The approach regards AI in finance as an issue of unlocking predictive and prescriptive capabilities that underpin strategic decision-making and create new value, not just mere automation.
Building the right foundation: Delivery structure and enterprise architecture
Successfully integrating AI into the finance function is not just about choosing the right technology; rather, it is about building a sound foundation that will lay the base for effective implementation and adoption. This requires a robust delivery structure with a well-defined enterprise architecture supportive of the organization's ambitions in AI.
Delivery structure:
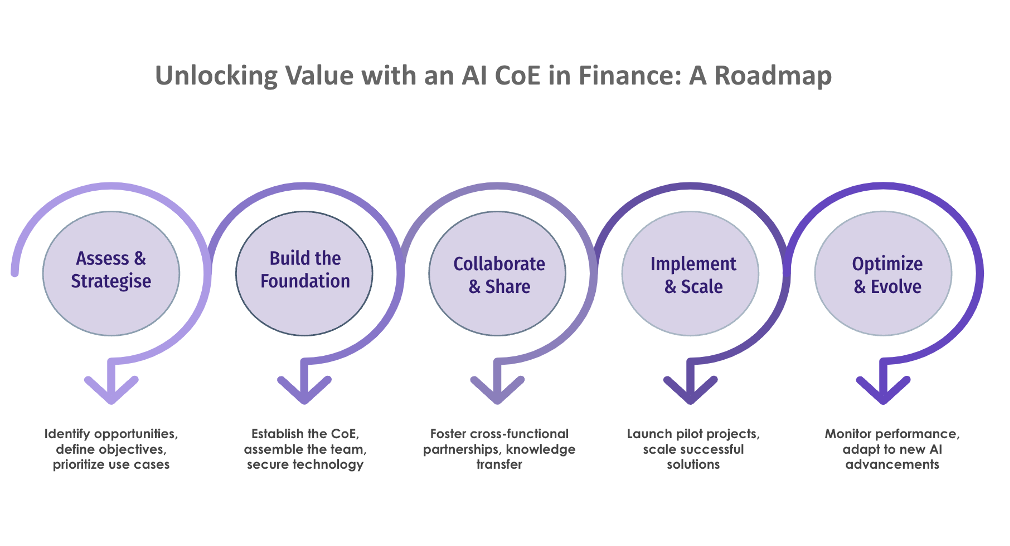
Well-structured collaboration, knowledge sharing, and effective execution are what organizations require for effective AI adoption.
The Finance Transformation CoE will act as a central hub of AI expertise to provide a crystal clear AI skillset to any organization by guiding and providing governance on best practices and support regarding AI initiatives across organizations. It drives innovation, aligns with overall business strategy, and inculcates a culture of data-driven decision-making.
Of equal importance is the formation of cross-functional teams comprising finance, IT, and operations, among others. In this way, it involves all the stakeholders in the designing and implementation of AI solutions so that the diverse needs within the organization are catered for.
Moreover, the usage of external expertise and technology partnerships would be an effective means of guaranteeing access to those particular skills and solutions that may be beyond reach or even unavailable internally. This, therefore, will help organizations hasten AI implementation and tap into state-of-the-art technologies and best practices.
Finally, this will be change management for successful AI adoption. It will be important to clearly communicate the process along with comprehensive training and sustained support to enable employees to navigate through changes resulting from AI and resolve their anxieties so as to work in a culture of continuous learning and improvement.
Enterprise architecture
A clearly defined enterprise architecture ensures seamless integration of the capabilities of AI across all functions of finance, including financial planning, accounting, treasury, risk management, tax, procurement, revenue management, reconciliation, and strategic finance. It shall guarantee that AI will be utilized to its fullest extent throughout the whole finance function, hence driving maximum impact on efficiency, decision-making, and risk management.
From the technical perspective of this architecture, it needs to have a unified data layer that allows the aggregation of data from various sources into one centralised repository and has quality control in data maintained uniformly.
The integration layer, via APIs and data pipelines, shall provide for efficient communication amongst the numerous systems involved that allow proper flow and, where applicable, analyses of the data. The AI and analytics layer provides the platform for creating and deploying AI models and advanced analytics. The application layer integrates the use of AI into existing financial applications to drive greater automation, insight, and decision-making.
The interface layer, including user-friendly dashboards, mobile apps, and conversational chatbots, will easily bring user access to AI-powered insights and tools by making interactions with data and systems intuitive.
Security measures and compliance frameworks are significant in the security and governance layer for protection of data and responsible, ethical use of AI systems. The infrastructure layer finally consists of scalable cloud and on-premise resources that enable AI workloads to scale systems for handling rapid growth in volume and complexity of financial data.
Governance of Data and AI
The only way organisations can avoid inefficiencies, overlaps, and silos is by ensuring a strong data and AI governance framework. While various departments may have their respective data and AI initiatives, it requires a more centralised governance structure to achieve consistency across the board and to encourage collaboration to maximise value from these efforts.
Finance can lead this cross-functional effort, given their intimate understanding of enterprise data management, regulatory compliance, financial reporting, reconciliation, and balance sheet management. It includes:
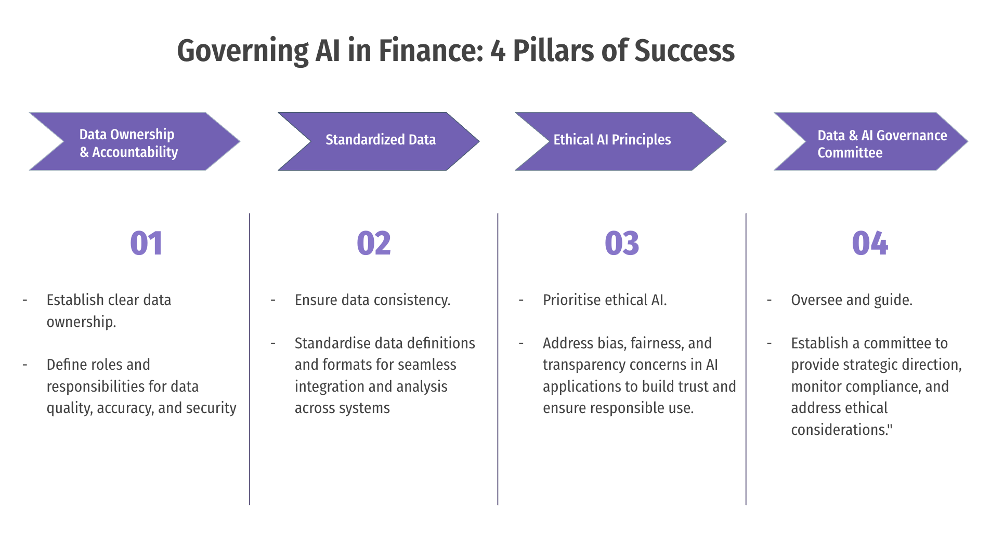
By embedding the governance of data and AI into its core functions, finance can encourage cross-functional cohesion, optimise the use of data, and unlock the full value of AI while minimising the risks of AI.
Reaping the rewards: Benefits and outcomes
Where organizations are able to effectively fold AI into their finance functions, they tend to achieve major dividends on efficiency, decision-making, risk management, and strategic positioning.
Automation and efficiency
AI-driven automation cuts out the tedium from routine operations, such as invoice processing, which often yields appreciable efficiency dividends.. This is the equivalent of a real cost saving; in one 2024 Deloitte case study, a global consumer goods company reduced finance operating costs by 15% through the use of AI-driven automation.
Decision making enhanced
Real-time analytics coupled with AI-cognitive-driven insight can drive data-driven decisions toward better financial performance. According to PwC’s Global NextGen survey in 2024, 70% of finance executives believe AI will significantly enhance forecasting accuracy. Indeed, in Unilever, its 2023 annual report showed that the AI-powered demand forecast enhanced the forecast accuracy by 20%, with obvious further effects on better inventory management and reduced waste.
Risk mitigation
AI enables risk management to achieve higher levels in terms of fraud detection, compliance monitoring, and risk assessment. It has also been noted that synthetic identity fraud increased by 47% in 2023, further cementing the requirement for fraud advanced detection systems powered by AI. In response to these issues, the use of AI in risk mitigation is growing.
According to Market.us, the AI In Fraud Detection Market valued $12.1 billion in 2023 is further expected to reach a market size of $108.3 billion by 2033 growing at a CAGR of 24.50%. It has grown rapidly as fraud activities they conduct are evolutionary in nature, and most organisations now need to deploy AI technologies inside fraud prevention solutions that ensure efficiency and accuracy.
Strategic partnership
AI enables finance to become a strategic partner in the business by bringing real-time insights with predictive capability. AI in the real estate sector helps partnerships study market trends, property values, and customer preferences. Predictive analytics can guide developers and investors in forming strategic alliances to invest in high-growth regions or projects.
In 2024, the global generative AI in real estate market size is calculated at USD 437.65 million, grew to USD 488.06 million in 2025, and is predicted to hit around USD 1,302.12 million by 2034, expanding at a CAGR of 11.52% between 2024 and 2034.
Competitive advantage
Early adoption of AI in finance positions organizations as innovators. Thus, early-bird entities can adapt to changing market conditions and outperform competitors. According to Gartner, by 2026, 90% of finance functions will deploy at least one AI-enabled technology solution, but less than 10% of functions will see headcount reductions.
These examples represent very concrete benefits of AI in finance from a variety of industries. Early adoption of AI in Finance places organizations in innovator positions, thus the ability to race ahead of others and respond to constantly changing market conditions. It allows the organization to be at an advantage by applying AI in its efficiencies, innovation, and customer service.
Future of finance: AI-driven and data-rich
The future of finance demands a commitment to change, strategic investment, and readiness to embrace transformation. To fully realize the potential of AI-driven finance, organizations need to foster active stakeholder engagement, invest in relevant talent, and continuously monitor and adapt their changes.
Key pillars of this transformation include: securing executive sponsorship, roadmapping, upskilling the finance team, and monitoring key performance indicators (KPIs) that assure desired outcomes from AI initiatives.
Sustainable finance
Beyond mere efficiency gains and enhanced decision-making, the future of finance will run on AI and big data analytics with a new focus on green and sustainable finance. Its practices would be enabled through the use of AI. Moreover, KPMG in its recent article also highlighted that AI is revolutionizing sustainable finance by enabling advanced risk assessment, climate risk modeling, impact investing, sustainable supply chain management, and greenwashing detection, empowering financial institutions to drive positive environmental and social change while maximizing returns.
However, widespread adoption is still hindered by challenges such as data availability, model accuracy, regulatory uncertainty, and varying levels of maturity across different sectors and organizations. This is not just a shift; it is a fundamental redefinition of how financial systems will operate in an increasingly focused on sustainability world.
Monetizing data and AI models
Data and AI models will evolve from being tools into crucial assets and intellectual property (IP) for companies. Accordingly, taking a lead interest in the development and deployment of innovative solutions with AI, new revenue streams for the finance functions could come from offering data-driven products and services, licensing AI models, or even creating data marketplaces.
The European Commission estimates that the EU’s data economy alone will be worth €829 bn in 2025, accounting for around 6% of regional GDP. In this new realm, the ability to use and commercialize data will become a key differentiator for forward-thinking financial companies.
Enhanced cybersecurity
In the wake of increased reliance on data and AI, the need for strong cybersecurity measures will be very crucial as finance functions. AI applications will be made to detect and prevent any form of cyber threat, which will be very crucial in keeping sensitive financial data and maintaining the integrity of the financial systems.
The market for AI-based cybersecurity is set to grow remarkably - from $24.3 billion in 2023 to nearly $134 billion by 2030, according to Statista. This surge underscores the crucial need for robust security measures as financial companies continue their digital presence and transformation.
While ransomware, in recent years, has attacked many sectors other than the financial sector, some of the major sectors involved are manufacturing, healthcare, and energy.
Transforming cybersecurity with AI
Discover how AI is transforming cybersecurity from both a defensive and adversarial perspective, featuring Palo Alto Networks’ CPO Lee Klarich.
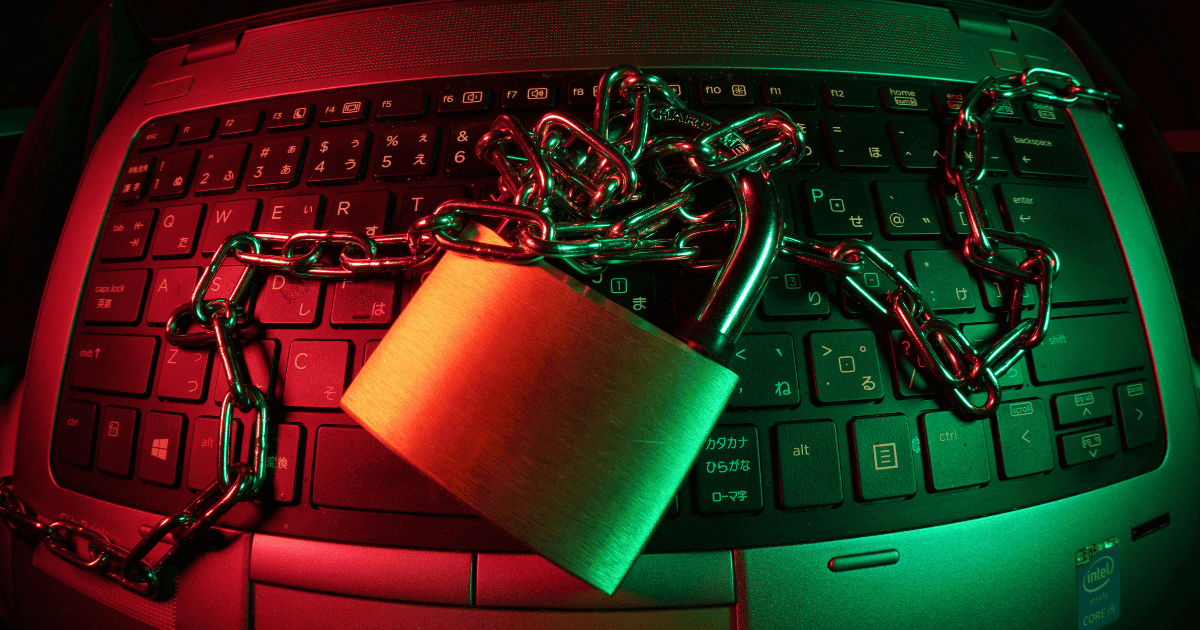
For example, in the Colonial Pipeline attack in 2021, both the financial and operational data were affected. Most businesses had to use AI to focus on spotting anomalies in network activity to quickly identify a breach. In manufacturing, ransomware attacks on supply chains have put companies into adopting AI-driven security systems that can predict vulnerabilities based on data patterns and guarantee protection for financial transactions and intellectual property.
Meanwhile, in healthcare, AI-powered cybersecurity solutions go into the protection of sensitive patient data and financial records after the ransomware attacks that struck hospital systems in 2024 in the US exposed patient and financial information.
Finance self-service agent assistants
Beyond these trends, the rise of AI-driven self-service agent assistants is about to revolutionize the way finance professionals interact with data. The assistants will use NLP to enable users to analyze, process, and manage financial data by using conversational language. Suppose you are asking an AI assistant to "forecast the revenue of next quarter based on current trends" or "identify any anomalies in this month's expenses." There is no need for complicated software products or specialized skills. In other words, everyone can use this highly effective tool for financial analysis.
For example, in 2022 Gartner predicted that by 2025 - 70% of white-collar workers will interact with conversational platforms daily. Additionally, the rise of AI has significantly accelerated the growth of self-service analytics, particularly by enhancing user accessibility and insight generation.
Gartner also predicts that by 2025, 80% of data and analytics innovations will be developed with AI and machine learning, further underscoring the pivotal role of AI in self-service analytics tools. These AI-driven assistants will not only respond to questions but will evolve into proactive advisors anticipating needs and automating routine tasks like report composition and account reconciliation.
AI-driven transformation in finance: What's next?
The finance function is on the verge of a profound transformation, with AI and data analytics becoming essential for driving operational efficiency, revenue assurance, cost optimization, risk mitigation, and strategic growth.
As we look to the future, financial leaders must embrace continuous learning, agile adaptation, and robust data governance to fully leverage the potential of AI. Organizations should prioritize building data-driven cultures, investing in cross-functional teams, and implementing advanced AI systems to maintain a competitive edge.
Emerging technologies such as generative AI, predictive analytics, and self-service AI-driven tools will revolutionize how finance teams operate. The next step of finance transformation will see these technologies more deeply into daily processes, enabling finance functions to evolve from reactive to proactive strategic partners. Furthermore, sustainable finance, enhanced cybersecurity, and the monetization of AI and data assets will become crucial focus areas.
For businesses, now is the time to invest in talent development, strategic AI roadmaps, and continuous monitoring of AI-driven initiatives. Those that do will gain a competitive edge, unlocking new efficiencies, revenue streams, and long-term value. As finance leaders, the opportunity is clear: embrace AI’s potential to redefine finance as a data-rich, AI-driven powerhouse for the future.
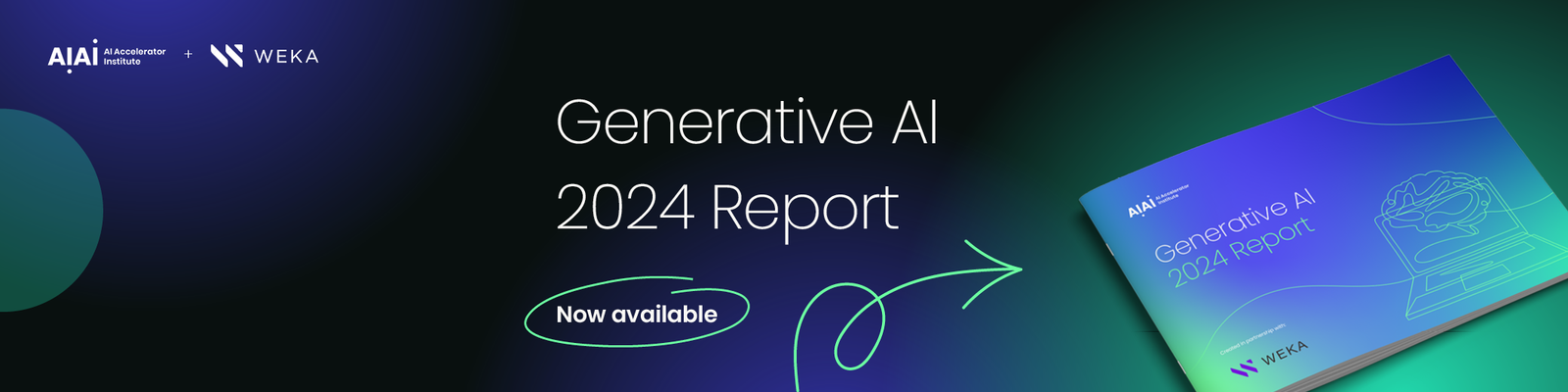
#000, #2022, #2023, #2024, #Accessibility, #Accounting, #Accounts, #Adoption, #Agent, #Agile, #Ai, #AIAdoption, #AiAssistant, #AIInFinance, #AIModels, #AISystems, #AIPowered, #Algorithms, #Analyses, #Analysis, #Analytics, #AnnualReport, #Anomalies, #AnomalyDetection, #APIs, #ApplicationLayer, #Applications, #Approach, #Apps, #Architecture, #Art, #Article, #Articles, #Artificial, #ArtificialIntelligence, #Assessment, #Assets, #Automation, #Bi, #BigData, #BigDataAnalytics, #Billion, #Board, #Breach, #Budgeting, #Building, #Bundle, #Business, #BusinessIntelligence, #CAS, #CaseStudy, #Change, #ChangeManagement, #Chatbots, #Climate, #Cloud, #Collaboration, #Collections, #Communication, #Communications, #Companies, #Complexity, #Compliance, #Composition, #Comprehensive, #Concrete, #Continuous, #ContinuousMonitoring, #CreditScoring, #Crime, #CrudeOil, #Crystal, #CustomerService, #Cyber, #CyberThreat, #Cybersecurity, #Data, #DataAnalysis, #DataAnalytics, #DataExtraction, #DataGovernance, #DataManagement, #DataMatching, #DataPipelines, #DataSources, #DataWarehouse, #DataWarehouses, #DataDriven, #DataDrivenDecisions, #Deal, #DecisionMaking, #Deloitte, #Deploying, #Deployment, #Detection, #Developers, #Development, #Driving, #Dynamics, #Economy, #Edge, #Effects, #Efficiency, #EmergingTechnologies, #Employed, #Employees, #Energy, #EnergyConsumption, #Engines, #Enterprise, #EnterpriseArchitecture, #EnterpriseResourcePlanning, #Enterprises, #Environment, #Environmental, #Erp, #Eu, #EuropeanCommission, #Evolution, #Executives, #Exercise, #ExpenseManagement, #ExpenseReporting, #Finance, #Financial, #FinancialCrime, #FinancialSector, #FinancialServices, #FinancialTransactions, #Focus, #Forecast, #Form, #Foundation, #Framework, #Fraud, #FraudDetection, #FraudPrevention, #Fraudsters, #Full, #Functions, #Fundamental, #Future, #Gartner, #Generative, #GenerativeAi, #Global, #Governance, #Green, #Growth, #Hand, #Health, #HealthCare, #Healthcare, #HighlyEffective, #History, #How, #Human, #HumanCapital, #Identity, #Impact, #Indeed, #IndustrialRevolution, #Industries, #Industry, #Infrastructure, #Innovation, #Innovations, #InSight, #Insights, #Integration, #IntellectualProperty, #Intelligence, #InventoryManagement, #Investing, #Investment, #Investments, #Invoice, #Invoices, #Invoicing, #IP, #Issues, #It, #Language, #LanguageModels, #LargeLanguageModels, #Learning, #LED, #LegacySystems, #Legislation, #LESS, #LLMs, #MachineLearning, #Management, #Manufacturing, #Mitigation, #Ml, #MlModel, #Mobile, #MobileApps, #Model, #Modeling, #Models, #Monitor, #Monitoring, #Nature, #Network, #Networks, #News, #Nlp, #Oil, #One, #OperationalEfficiency, #Optimization, #Oracle, #Organization, #Organizations, #Other, #PaloAltoNetworks, #Paper, #Partners, #Partnerships, #Patterns, #Payments, #Performance, #Pipelines, #Planning, #Platform, #Play, #Policies, #Pos, #Positioning, #Power, #Predictions, #PredictiveAnalytics, #Prevent, #Prevention, #Pricing, #Proactive, #Process, #Productivity, #Pwc, #Quantum, #QuantumLeap, #Ransomware, #RansomwareAttacks, #RealEstate, #RealTime, #ReALM, #Recording, #Regulation, #Regulations, #RegulatoryCompliance, #Reliance, #Report, #Reports, #Repository, #Resilience, #Resource, #ResourceAllocation, #Resources, #Retail, #Revenue, #Risk, #RiskAssessment, #RiskManagement, #Risks, #Robotic, #RoboticProcessAutomation, #Rpa, #Rules, #Sap, #Scalable, #Scale, #Scores, #SEC, #Securing, #Security, #SelfServiceAnalytics, #Sensitive, #Skills, #Social, #Software, #Solve, #Sound, #Standards, #Statistics, #Strategy, #Streams, #Stress, #Structure, #Study, #SupplyChain, #SupplyChainManagement, #SupplyChains, #Sustainability, #Sustainable, #Talent, #Tax, #Teams, #Technology, #Telecom, #Telecommunications, #Threats, #Time, #Tool, #Tools, #Training, #Transaction, #Transformation, #Transparency, #Trends, #Unified, #UnstructuredData, #Upskilling, #Vendor, #Visibility, #Vulnerabilities, #Walmart, #Warehouses, #Waste, #Work, #Workflow, #World
Published on The Digital Insider at https://is.gd/wjtxqv.
Comments
Post a Comment
Comments are moderated.