The tremendous potential of digital twin technology – with its ability to create digital replicas of physical objects, processes and environments – has applications that span across industries, from replicating hazardous environments to displaying spacecrafts for remote training purposes. Recent analysis from McKinsey suggests the interest is so profound that the global market for digital twins will grow about 60% per year over the next five years and reach $73.5 billion by 2027. The interest is clearly there, but has adoption really followed?
The answer – it's complicated. Digital twin technology and its use cases have evolved immensely, but challenges must be addressed for digital twins to be adopted at scale.
The Evolution of Digital Twins
True adoption of digital twin technology has been slow because, until recently, it lacked the intelligence to go beyond simply representing an asset. More valuable would be the ability to accurately simulate, predict, and control its behavior. Digital twins were also bespoke and lacked the ability to learn globally from the behavior of similar assets. Their insights were siloed and not always applicable to broader organizational needs, making them a hefty investment with narrow returns.
Even so, some early adopters of digital twins include the manufacturing, retail, healthcare, and automotive industries, which have been able to test new facilities, configurations, and processes in a controlled environment.
With new AI-driven approaches, we will see a rapid shift from “digital twins” to AI-powered “simulation” and “agency” that will dramatically broaden the use cases and drive widespread adoption. Let’s look at these categories of use:
- Representation – The early iterations of digital twins were simple digital representations of assets, which were not particularly useful beyond select niche use cases for improving the design and execution of certain tasks. In essence, this is the “replica” state of digital twin technology.
- Simulation – Today, digital twins are evolving from representation to simulation, which benefits a wider set of use cases. Simulation means that digital twins are not only mirroring the asset or environment, but are also accurately simulating future scenarios. In this stage, they are able to learn from data from other similar processes to garner meaningful insights. Simulation twins use AI algorithms to simulate production outcomes, recommend optimal machine settings, and guide production teams toward improved business objectives in a manufacturing setting.
- Agency – The next evolution after simulation will be agency, which will enable assets, processes, and entire parts of production to plan and act autonomously. In this stage, they will also make complex decisions and work in partnership with people to drive more sustainable production. This is the digital twin agent stage.
Moving between stages requires different levels of supporting technology, and it is paramount that organizations have the right tech stack to achieve the maximum impact and ROI of digital twins.
Foundational Technology for Digital Twins
The right foundational technology must be in place before moving from representation to simulation and then, ultimately, agency.
Using manufacturing as an example again, organizations that want to create a digital simulation of a given process or factory environment must have reliable online sensing capabilities. These sensors feed data from the input and output at various critical stages of the journey in order to provide robust insights to inform a simulation. A lot of this data is readily available, and we have seen process manufacturers with quality online measurements on the outputs (i.e., paper), but there is usually a gap in sensing measurements for the inputs (i.e., wood fibers that go into paper pulp production).
To circumvent this, manufacturing teams must clearly define the simulation they are trying to achieve and the various inputs, machines, and systems that are involved, along with the different parameters of each stage throughout the process. This likely requires tapping experts across multiple functions to ensure all aspects of the model are accounted for, which will then help ensure the data is robust enough to power a simulation.
Connectivity and Comparison
Digital twins that are completely isolated are missing out on learnings from other models in similar scenarios. The models contributing to the digital twin themselves need to be fed with data from other similar models and digital twins to demonstrate what “great” or optimal looks like globally, not just within the local process that is being examined.
As a result, digital twins require a large cloud component, or else organizations risk losing out on any semblance of the full promise this technology offers.
The other side of the coin is that digital twins must not rely solely on cloud technology because the latency of the cloud can create obstacles for factors like collecting real-time data and real-time instructions. Consider how pointless it would be to have a simulation intended to prevent machine failures only for the simulation to detect a broken belt well after the piece has stopped functioning properly and the entire machine is at a standstill.
To overcome these challenges, it may be wise to add a component that is edge-AI-enabled. This ensures data can be captured as close as possible to the process being simulated.
Possible Pain Points with Deployment and Management
In addition to having the right tech stack and infrastructure to capture the necessary data for AI-powered simulation twins, trust remains a significant roadblock to deployment. Taxi drivers in London may know the city map and all its shortcuts, but GPS typically equips drivers with more accurate routes by factoring in traffic data. Similarly, engineers and manufacturing professionals need to experience accurate and safe simulations to fully gain confidence in their capabilities.
Gaining trust takes time, but transparency with the models and with the data feeding the digital twins can speed up this process. Organizations should think strategically about the mindset shift that is necessary to get teams to trust the insights from this powerful technology – or risk missing out on ROI.
The Road to Agency
Despite the promise of digital twins, adoption has been relatively slow–until recently. The introduction of AI-powered models can take digital twins from representation to simulation by connecting insights from other models to build off unique learnings.
As investment and trust increase, digital twins will eventually reach agency status and be able to make complex decisions on their own. The true value has yet to be unlocked, but digital twins have the potential to transform industries from manufacturing to healthcare to retail.
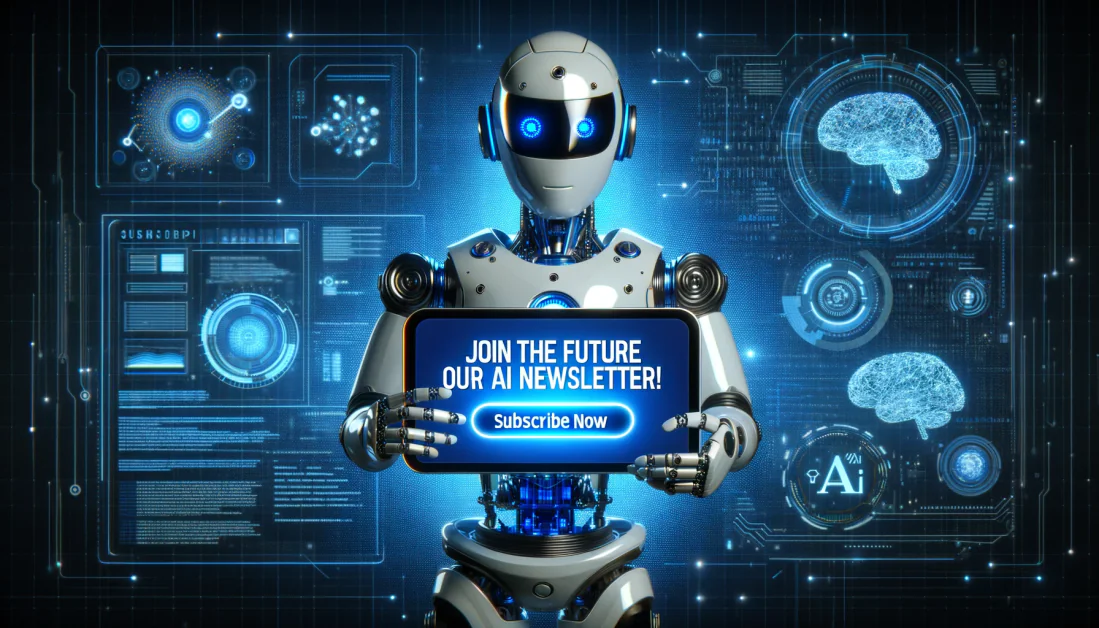
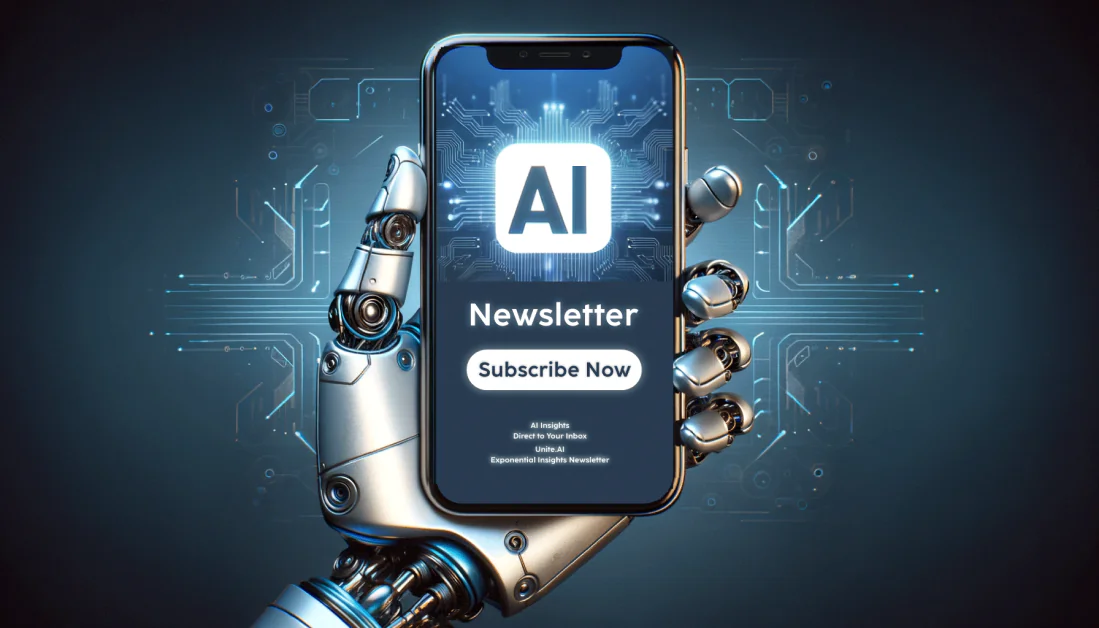
#ADD, #Adoption, #Agent, #Ai, #AIPowered, #Algorithms, #Analysis, #Applications, #Assets, #Augury, #Automotive, #Behavior, #Billion, #Business, #Capture, #Cloud, #CloudTechnology, #Connectivity, #Data, #Deployment, #Design, #DigitalReplicas, #DigitalTwins, #Edge, #Engineers, #Environment, #Evolution, #Facilities, #Fibers, #Full, #Functions, #Future, #Gap, #Global, #GPS, #Healthcare, #How, #Impact, #Industries, #Infrastructure, #Insights, #Intelligence, #Investment, #It, #Latency, #Learn, #London, #Manufacturers, #Manufacturing, #Map, #Measurements, #Model, #Models, #Objects, #Organizations, #Other, #Pain, #Paper, #Partnership, #Plan, #Power, #Prevent, #Process, #Production, #RealTime, #RealTimeData, #Remote, #Retail, #Risk, #ROI, #Scale, #Sensing, #Sensors, #Shortcuts, #Simulation, #Simulations, #Speed, #Stack, #Sustainable, #Taxi, #Teams, #Tech, #TechStack, #Technology, #ThoughtLeaders, #Time, #Training, #Transform, #Transparency, #Trust, #Wood, #Work
Published on The Digital Insider at https://is.gd/TckENW.
Comments
Post a Comment
Comments are moderated.