As has been the case with numerous technologies before it, artificial intelligence (AI) is being hailed as the next great innovation enterprises simply must use. Ironically, the underlying technology has been around for decades, but with the latest iterations, the hype has reached a fever pitch—outpacing the reality of implementation across the enterprise. Yet, as IT teams face increasing pressure to get on board the IT train, they must balance that enthusiasm with the reality of the bottom line. Different implementations require different levels of investment, meaning they must also yield a different return—often on a different timetable.
The ability to deliver successful AI products depends on numerous factors: specific strategies, planning and execution chosen by business leaders; availability of skilled resources; fit within product roadmap; organizational acceptance of risk; and time management against expected return on investment (ROI).
Balancing these factors is the challenge, but following these three steps can keep organizations on the path toward AI ROI.
Understand the Technology
Many enterprises enter the AI fray believing they are behind but not fully understanding why, how, or even what the technology is. As a result, their first task is distinguishing among different flavors of AI, beginning with precision AI vs. generative AI.
Precision AI is the use of machine learning and deep learning models to improve outcomes. It enables enterprises to automate decision-making processes, creating efficiencies and increasing ROI. Precision AI has matured into an established workhorse technology for enterprises that continues to see significant adoption and is becoming more mainstream by the day.
Generative AI (GenAI) is new and has risen to prominence since OpenAI released ChatGPT in late 2022. Consisting of foundational large language models (LLMs) trained with billions of parameters to generate new semantic text context, GenAI offers significant opportunities for business impact and operational efficiency but it’s early in its adoption lifecycle.
One significant hurdle is the standard for data quality, which is elevated for GenAI applications since low-quality datasets can introduce transparency and ethical issues.
Data reliability begins with designing and implementing workflows; establishing pipelines to perform; abstracting through APIs; curating and democratizing; and processing different data types. Rather than the previous generation of data quality requirements that included the 4Vs (volume, velocity, veracity and variety), AI needs new requirements that include 4Ps: prediction, productivity, precision, and persona at scale.
Prediction: AI algorithms allow the use of statistical analysis to find patterns in the data and identify behaviors to predict and forecast future events by correlating historical data at rest and data streaming to make decisions in real-time.
Productivity: AI enables business process automation, which increases enterprise operational efficiency and productivity, reducing repetitive tasks and freeing up staff time to work on more strategic assignments.
Precision: This metric measures the model results in a way that machine learning models can produce accuracy between acceptable range determined by the use cases. Precision is also calculated as the number of true positives divided by total number of positive predictions.
Persona at scale: This refers to the process of using reliable data such as customer purchase histories, on-site actions, customers’ sentiment analysis for specific products and survey responses. It delivers individualized experiences across demographics.
In addition to data quality, enterprises must consider numerous other factors—both internal and external—when evaluating their AI readiness: governance, compliance alignment, cloud investments, talent, new business operations models, risk management, and leadership commitment.
Organizations must begin by establishing an AI vision that matches their goals and strategic objectives. Buy-in from the C-suite is critical, as AI deployments require significant up-front investment. The CIO must clearly articulate the path to ROI to the entire C-suite—a true test of the CIO in elevating IT from an enabling function to a strategic one.
Next, the organization must align people, processes, and technology. AI requires new skills and certifications such as deep learning models and machine learning, as organizations have traditionally integrated AI into human workflows. However, GenAI reverses the dynamic, but most best practices and responsible use guidelines still include a “human in the loop” component to maintain ethical standards and values.
An AI deployment also demands new business processes for governance and data quality assurance, enabling the data scientists responsible for delivering new AI models to solve complex business problems.
As new AI products are designed, developed, and manufactured for production, enterprises must also remain vigilant of the AI industry’s latest regulatory policies. The European AI act has established best practices for using AI—and consequences for not following those policies. As a result, enterprises have constructed teams to create, evaluate and update efforts around AI regulations.
With enterprises becoming increasingly data-driven, they must develop foundational strategies to protect the data assets enabling them to deliver the best insights through analytics process automation platforms. From there, they can select the AI technologies and new platforms that make the most sense for them.
Define the Business Case
Finally, true return on an AI investment requires selling the benefit to customers, meaning AI readiness requires a new business mindset as the technology is driving transformation for enterprises across industries.
Successful AI product development requires an intimate understanding of industry-specific customer journeys and aligning AI solutions with business objectives. Customer centricity plays a key role in developing new operating models, and modern technologies are used to increase efficiency.
For instance, customers looking for small wins in AI maturity can rely on their software assets and cloud infrastructure to develop new products and solutions. This keeps satisfaction among employees higher and maintains their focus on exceeding customer expectations.
That said, the core of the organization should focus on shortening time-to-market and improving new process management to shorten the product development life cycle and increase the efficiency of delivering new products. For example, a distributed augmented data analytics platform is used to automate the ingestion, curation, democratization, processing, and analytics in real-time—all of which increase productivity and ROI.
Unlock the Full Potential of AI ROI
AI at its core stands for advanced algorithms, data quality, computing power, Infrastructure as Code, governance, responsible AI with ethics to protect data privacy and confidentiality. The essentials of AI application readiness and the challenges of data management require hardness data-driven frameworks, people, process, strategy ethics and technology platforms.
Concurrently, Mckinsey reports that 65% of enterprises are using AI technologies—double the number from last year. It demonstrates momentum, but deployments are still moving slowly from curiosity to real business use cases at scale. GenAI is delivering new breakthroughs, enabling organizations to harness new capabilities through the development of semantic and multi-modal LLMs. It democratizes a full spectrum of AI capabilities, enabling them to generate new revenue streams.
With the right strategy, leadership commitment, and investment in the correct use cases, businesses can gain significant value and drive transformative growth through AI.
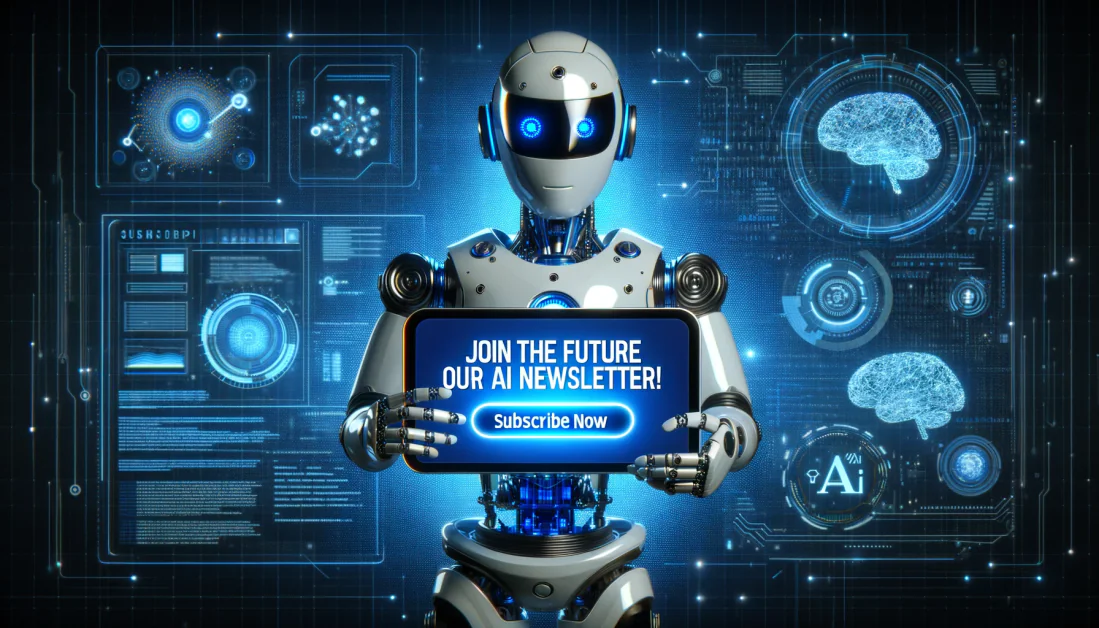
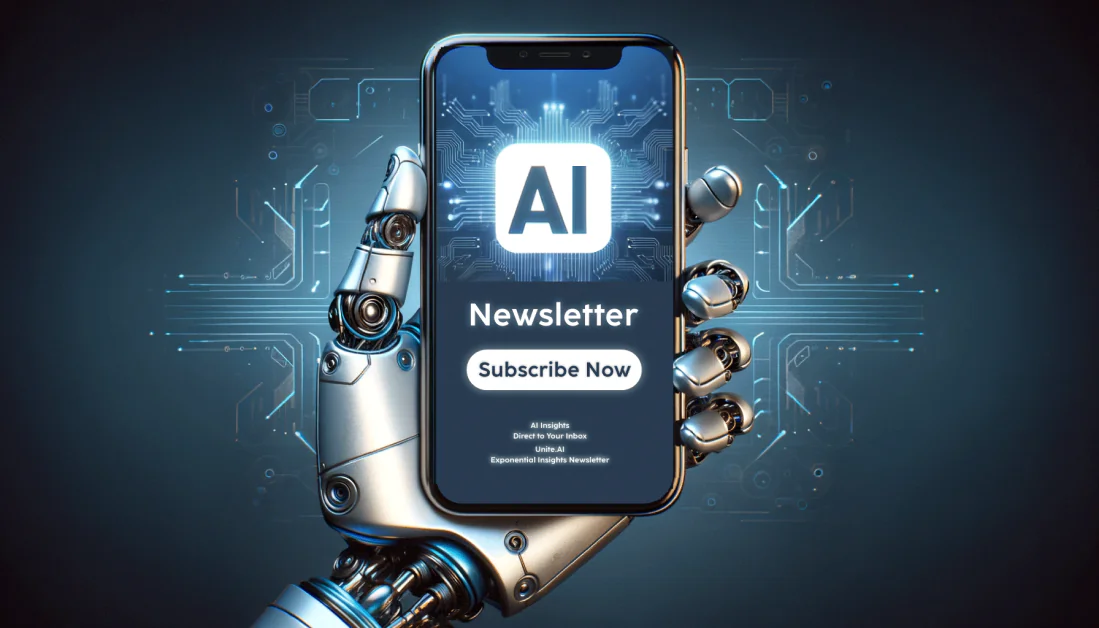
#2022, #Adoption, #Ai, #AiAct, #AIModels, #Algorithms, #Analysis, #Analytics, #APIs, #Applications, #Artificial, #ArtificialIntelligence, #Assets, #Automation, #Board, #Business, #CSuite, #Certifications, #Challenge, #ChatGPT, #Cio, #Cloud, #CloudInfrastructure, #Code, #Compliance, #Computing, #Curiosity, #Data, #DataAnalytics, #DataManagement, #DataPrivacy, #DataQuality, #DataStreaming, #DataDriven, #Datasets, #DeepLearning, #Democratization, #Deployment, #Development, #Double, #Driving, #Efficiency, #Employees, #Enterprise, #Enterprises, #Ethics, #Events, #Focus, #Forecast, #Full, #Future, #Genai, #Generative, #GenerativeAi, #Governance, #Growth, #Guidelines, #How, #Human, #Impact, #Industries, #Industry, #Infrastructure, #Innovation, #Insights, #Intelligence, #Investment, #Investments, #Issues, #It, #Language, #LanguageModels, #LargeLanguageModels, #Leadership, #Learning, #Lenovo, #Life, #LLMs, #Loop, #MachineLearning, #Management, #Model, #Models, #MultiModal, #One, #Openai, #OperationalEfficiency, #Organization, #Organizations, #Other, #Patterns, #Pipelines, #Planning, #Platform, #Policies, #Power, #Predictions, #Privacy, #Process, #ProcessManagement, #Production, #Productivity, #RealTime, #Regulations, #Reliability, #Reports, #Resources, #ResponsibleAI, #REST, #Revenue, #Risk, #RiskManagement, #ROI, #Scale, #SentimentAnalysis, #Skills, #Software, #Solve, #Spectrum, #Staff, #Standards, #Strategy, #Streaming, #Streams, #Talent, #Teams, #Technology, #Text, #ThoughtLeaders, #Time, #TimeManagement, #Transformation, #Transparency, #Vision, #Vs, #Work, #Workflows
Published on The Digital Insider at https://is.gd/7XR6zp.
Comments
Post a Comment
Comments are moderated.