The Ethical Minefield of AI Scaling: Building Trustworthy AI for Large-Scale Deployments | By The Digital Insider
A few years ago, a tutoring company paid a hefty legal settlement after its artificial intelligence powered recruiting software disqualified over 200 applicants based solely on their age and gender. In another case, an AI recruiting tool down-ranked women applicants by associating gender-related terminology with underqualified candidates. The algorithm amplified hiring biases at scale by absorbing historical data.
Such real world examples underscore the existential risks for global organizations deploying unchecked AI systems. Embedding discriminatory practices into automated processes is an ethical minefield jeopardizing hard earned workplace equity and brand reputation across cultures.
As AI capabilities grow exponentially, business leaders must implement rigorous guardrails including aggressive bias monitoring, transparent decision rationale, and proactive demographic disparity audits. AI can’t be treated as an infallible solution; it is a powerful tool that demands immense ethical oversight and alignment with fairness values.
Mitigating AI Bias: A Continuous Journey
Identifying and correcting unconscious biases within AI systems is an ongoing challenge, especially when dealing with vast and diverse datasets. This requires a multifaceted approach rooted in robust AI governance. First, organizations must have full transparency in their AI algorithms and training data. Conducting rigorous audits to assess representation and pinpoint potential discrimination risks is critical. But bias monitoring cannot be a one-time exercise – it requires continuous evaluation as models evolve.
Let’s look at the example of New York City, which enacted a new law last year that mandates city employers to conduct annual third-party audits of any AI systems used for hiring or promotions to detect racial or gender discrimination. These ‘bias audit’ findings are publicly published, adding a new layer of accountability for human resources leaders when selecting and overseeing AI vendors.
However, technical measures alone are insufficient. A holistic debiasing strategy comprising operational, organizational, and transparency elements is vital. This includes optimizing data collection processes, fostering transparency into AI decision making rationale, and leveraging AI model insights to refine human-driven processes.
Explainability is key to fostering trust by providing clear rationale that lays bare the decision-making process. A mortgage AI should spell out exactly how it weighs factors like credit history and income to approve or deny applicants. Interpretability takes this a step further, illuminating the under-the-hood mechanics of the AI model itself. But true transparency goes beyond opening the proverbial black box. It’s also about accountability – owning up to errors, eliminating unfair biases, and giving users recourse when needed.
Involving multidisciplinary experts, such as ethicists and social scientists, can further strengthen the bias mitigation and transparency efforts. Cultivating a diverse AI team also amplifies the ability to recognize biases affecting under-represented groups and underscoring the importance of promoting inclusive workforce.
By adopting this comprehensive approach to AI governance, debiasing, and transparency, organizations can better navigate the challenges of unconscious biases in large-scale AI deployments while fostering public trust and accountability.
Supporting the Workforce Through AI’s Disruption
AI automation promises workforce disruption on par with past technological revolutions. Businesses must thoughtfully reskill and redeploy their workforce, investing in cutting-edge curriculum and making upskilling central to AI strategies. But reskilling alone is not enough.
As traditional roles become obsolete, organizations need creative workforce transition plans. Establishing robust career services – mentoring, job placement assistance and skills mapping – can help displaced employees navigate systemic job shifts.
Complementing these human-centric initiatives, businesses should enact clear AI usage guidelines. Organizations must focus on enforcement and employee education around ethical AI practices. The path forward involves bridging the leadership’s AI ambitions with workforce realities. Dynamic training pipelines, proactive career transition plans, and ethical AI principles are building blocks that can position companies to survive disruption and thrive in the increasingly automated world.
Striking the Right Balance: Government’s Role in Ethical AI Oversight
Governments must establish guardrails around AI upholding democratic values and safeguarding citizen rights including robust data privacy laws, prohibition on discriminatory AI, transparency mandates, and regulatory sandboxes incentivizing ethical practices. But excessive regulation may stifle the AI revolution.
The path forward lies in striking a balance. Governments should foster public-private collaboration and cross-stakeholder dialogue to develop adaptive governance frameworks. These should focus on prioritizing key risk areas while providing flexibility for innovation to flourish. Proactive self-regulation within a co-regulatory model could be an effective middle ground.
Fundamentally, ethical AI hinges on establishing processes for identifying potential harm, avenues for course correction, and accountability measures. Strategic policy fosters public trust in AI integrity but overly prescriptive rules will struggle to keep pace with the speed of breakthroughs.
The Multidisciplinary Imperative for Ethical AI at Scale
The role of ethicists is defining moral guardrails for AI development that respect human rights, mitigate bias, and uphold principles of justice and equity. Social scientists lend crucial insights into AI’s societal impact across communities.
Technologists are then charged with translating the ethical tenets into pragmatic reality. They design AI systems aligned with defined values, building in transparency and accountability mechanisms. Collaborating with ethicists and social scientists is key to navigate tensions between ethical priorities and technical constraints.
Policymakers operate at the intersection, crafting governance frameworks to legislate ethical AI practices at scale. This requires ongoing dialogue with technologists and cooperation with ethicists and social scientists.
Collectively, these interdisciplinary partnerships facilitate a dynamic, self-correcting approach as AI capabilities evolve rapidly. Continuous monitoring of real-world impact across domains becomes imperative, feeding back into updated policies and ethical principles.
Bridging these disciplines is far from straightforward. Divergent incentives, vocabulary gaps, and institutional barriers can hinder cooperation. But overcoming these challenges is essential for developing scalable AI systems that uphold human agency for technological progress.
To sum up, eliminating AI bias isn’t merely a technical hurdle. It’s a moral and ethical imperative that organizations must embrace wholeheartedly. Leaders and brands simply cannot afford to treat this as an optional box to check. They must ensure that AI systems are firmly grounded in the bedrock of fairness, inclusivity, and equity from ground up.
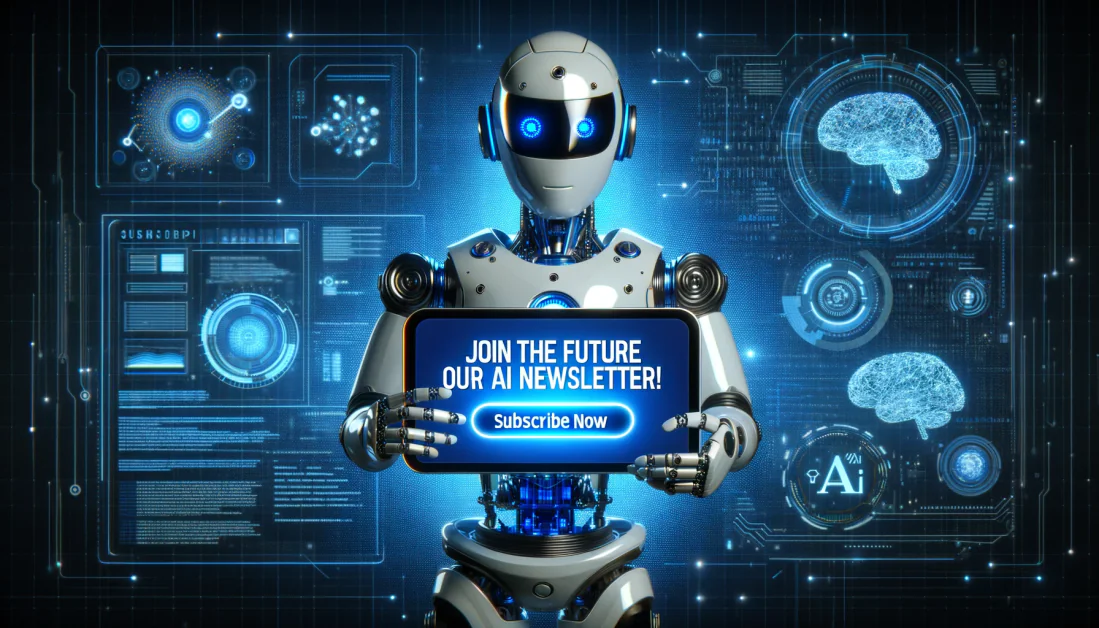
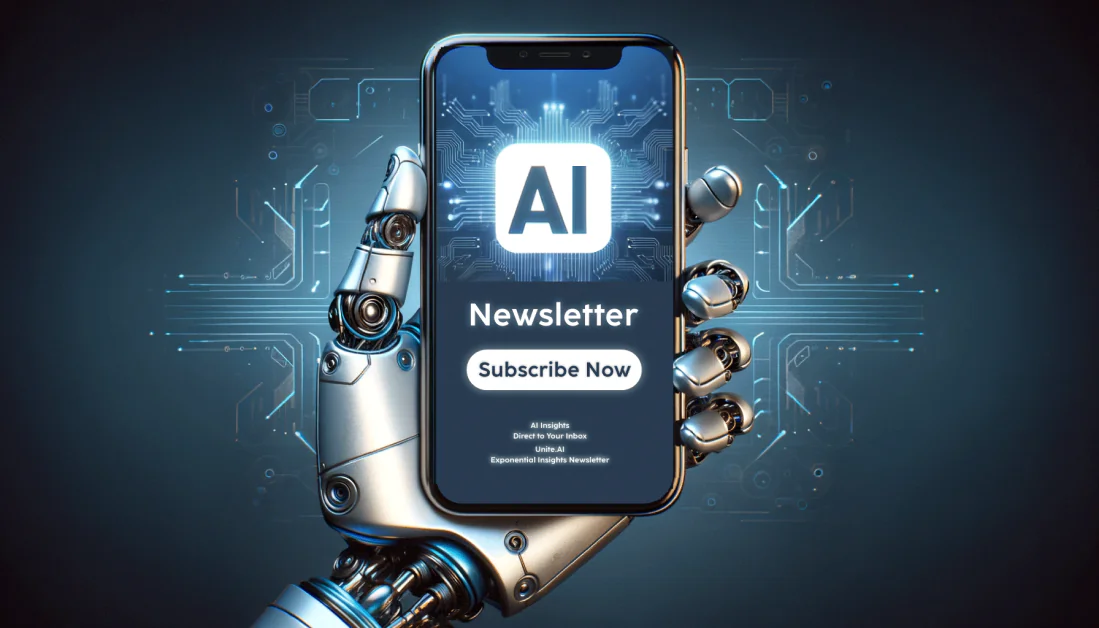
#Ai, #AIBias, #AIDevelopment, #AIEthics, #AiModel, #AISystems, #Algorithm, #Algorithms, #Approach, #Artificial, #ArtificialIntelligence, #Audit, #Automation, #Bias, #BlackBox, #Box, #Brands, #Building, #Business, #Career, #Challenge, #Collaboration, #Companies, #Comprehensive, #Continuous, #Course, #Cutting, #Data, #DataCollection, #DataPrivacy, #Datasets, #DecisionMaking, #Democratic, #Deploying, #Design, #Development, #Disruption, #Domains, #Edge, #Education, #EmployeeEducation, #Employees, #Equity, #EthicalAi, #Ethics, #Exercise, #Explainability, #Focus, #Full, #Gender, #Giving, #Global, #Governance, #Government, #Guidelines, #Hiring, #History, #How, #Human, #HumanResources, #HumanRights, #Impact, #Innovation, #Insights, #Intelligence, #Interpretability, #Investing, #It, #Justice, #Law, #Leadership, #Legal, #Mechanics, #Mentoring, #Mitigate, #Mitigation, #Model, #Models, #Monitoring, #NewYork, #NewYorkCity, #One, #Organizations, #PAID, #Partnerships, #Pinpoint, #Pipelines, #Placement, #Policies, #Policy, #Privacy, #Proactive, #Process, #Recruiting, #RecruitingSoftware, #Regulation, #Reskilling, #Resources, #Risk, #Risks, #Roles, #Rules, #Scale, #Scaling, #Skills, #Social, #Software, #Speed, #Strategy, #ThirdParty, #ThoughtLeaders, #Time, #Tool, #Training, #Transition, #Transparency, #Trust, #Upskilling, #Vendors, #Workforce, #Workplace
Published on The Digital Insider at https://is.gd/hxX69Q.
Comments
Post a Comment
Comments are moderated.