How seriously should governments take the threat of existential risk from AI, given the lack of consensus among researchers? On the one hand, existential risks (x-risks) are necessarily somewhat speculative: by the time there is concrete evidence, it may be too late. On the other hand, governments must prioritize — after all, they don’t worry too much about x-risk from alien invasions.
This is the first in a series of essays laying out an evidence-based approach for policymakers concerned about AI x-risk, an approach that stays grounded in reality while acknowledging that there are “unknown unknowns”.
In this first essay, we look at one type of evidence: probability estimates. The AI safety community relies heavily on forecasting the probability of human extinction due to AI (in a given timeframe) in order to inform decision making and policy. An estimate of 10% over a few decades, for example, would obviously be high enough for the issue to be a top priority for society.
Our central claim is that AI x-risk forecasts are far too unreliable to be useful for policy, and in fact highly misleading.
If the two of us predicted an 80% probability of aliens landing on earth in the next ten years, would you take this possibility seriously? Of course not. You would ask to see our evidence. As obvious as this may seem, it seems to have been forgotten in the AI x-risk debate that probabilities carry no authority by themselves. Probabilities are usually derived from some grounded method, so we have a strong cognitive bias to view quantified risk estimates as more valid than qualitative ones. But it is possible for probabilities to be nothing more than guesses. Keep this in mind throughout this essay (and more broadly in the AI x-risk debate).
If we predicted odds for the Kentucky Derby, we don’t have to give you a reason — you can take it or leave it. But if a policymaker takes actions based on probabilities put forth by a forecaster, they had better be able to explain those probabilities to the public (and that explanation must in turn come from the forecaster). Justification is essential to legitimacy of government and the exercise of power. A core principle of liberal democracy is that the state should not limit people's freedom based on controversial beliefs that reasonable people can reject.
Explanation is especially important when the policies being considered are costly, and even more so when those costs are unevenly distributed among stakeholders. A good example is restricting open releases of AI models. Can governments convince people and companies who stand to benefit from open models that they should make this sacrifice because of a speculative future risk?
The main aim of this essay is analyzing whether there is any justification for any of the specific x-risk probability estimates that have been cited in the policy debate. We have no objection to AI x-risk forecasting as an academic activity, and forecasts may be helpful to companies and other private decision makers. We only question its use in the context of public policy.
There are basically only three known ways by which a forecaster can try to convince a skeptic: inductive, deductive, and subjective probability estimation. We consider each of these in the following sections. All three require both parties to agree on some basic assumptions about the world (which cannot themselves be proven). The three approaches differ in terms of the empirical and logical ways in which the probability estimate follows from that set of assumptions.
Most risk estimates are inductive: they are based on past observations. For example, insurers base their predictions of an individual’s car accident risk on data from past accidents about similar drivers. The set of observations used for probability estimation is called a reference class. A suitable reference class for car insurance might be the set of drivers who live in the same city. If the analyst has more information about the individual, such as their age or the type of car they drive, the reference class can be further refined.
For existential risk from AI, there is no reference class, as it is an event like no other. To be clear, this is a matter of degree, not kind. There is never a clear “correct” reference class to use, and the choice of a reference class in practice comes down to the analyst’s intuition.
The accuracy of the forecasts depends on the degree of similarity between the process that generates the event being forecast and the process that generated the events in the reference class, which can be seen as a spectrum. For predicting the outcome of a physical system such as a coin toss, past experience is a highly reliable guide. Next, for car accidents, risk estimates might vary by, say, 20% based on the past dataset used — good enough for insurance companies.
Further along the spectrum are geopolitical events, where the choice of reference class gets even fuzzier. Forecasting expert Philip Tetlock explains: “Grexit may have looked sui generis, because no country had exited the Eurozone as of 2015, but it could also be viewed as just another instance of a broad comparison class, such as negotiation failures, or of a narrower class, such as a nation-states withdrawing from international agreements or, narrower still, of forced currency conversions.” He goes on to defend the idea that even seeming Black Swan events like the collapse of the USSR or the Arab Spring can be modeled as members of reference classes, and that inductive reasoning is useful even for this kind of event.
In Tetlock’s spectrum, these events represent the “peak” of uniqueness. When it comes to geopolitical events, that might be true. But even those events are far less unique than extinction from AI. Just look at the attempts to find reference classes for AI x-risk: animal extinction (as a reference class for human extinction), past global transformations such as the industrial revolution (as a reference class for socioeconomic transformation from AI), or accidents causing mass deaths (as a reference class for accidents causing global catastrophe). Let’s get real. None of those tell us anything about the possibility of developing superintelligent AI or losing control over such AI, which are the central sources of uncertainty for AI x-risk forecasting.
To summarize, human extinction due to AI is an outcome so far removed from anything that has happened in the past that we cannot use inductive methods to “predict” the odds. Of course, we can get qualitative insights from past technical breakthroughs as well as past catastrophic events, but AI risk is sufficiently different that quantitative estimates lack the kind of justification needed for legitimacy in policymaking.
In Conan Doyle’s The Adventure of the Six Napoleons— spoiler alert! — Sherlock Holmes announces before embarking on a stakeout that the probability of catching the suspect is exactly two-thirds. This seems bewildering — how can anything related to human behavior be ascribed a mathematically precise probability?
It turns out that Holmes has deduced the underlying series of events that gave rise to the suspect’s seemingly erratic observed behavior: the suspect is methodically searching for a jewel that is known to be hidden inside one of six busts of Napoleon owned by different people in and around London. The details aren’t too important, but the key is that neither the suspect nor the detectives know which of the six busts it is in, and everything else about the suspect’s behavior is (assumed to be) entirely predictable. Hence the precisely quantifiable uncertainty.
The point is that if we have a model of the world that we can rely upon, we can estimate risk through logical deduction, even without relying on past observations. Of course, outside of fictional scenarios, the world isn’t so neat, especially when we want to project far into the future.
When it comes to x-risk, there is an interesting exception to the general rule that we don’t have deductive models — asteroid impact. A combination of inductive and deductive risk estimation does allow us to estimate the probability of x-risk, only because we’re talking about a purely physical system. Let’s take a minute to review how this works, because it’s important to recognize that the methods are not generalizable to other types of x-risk.
The key is being able to model the relationship between the size of the asteroid (more precisely, the energy of impact) and the frequency of impact. Since we have observed thousands of small impacts, we can extrapolate to infer the frequency of large impacts that have never been directly observed. We can also estimate the threshold that would cause global catastrophe.
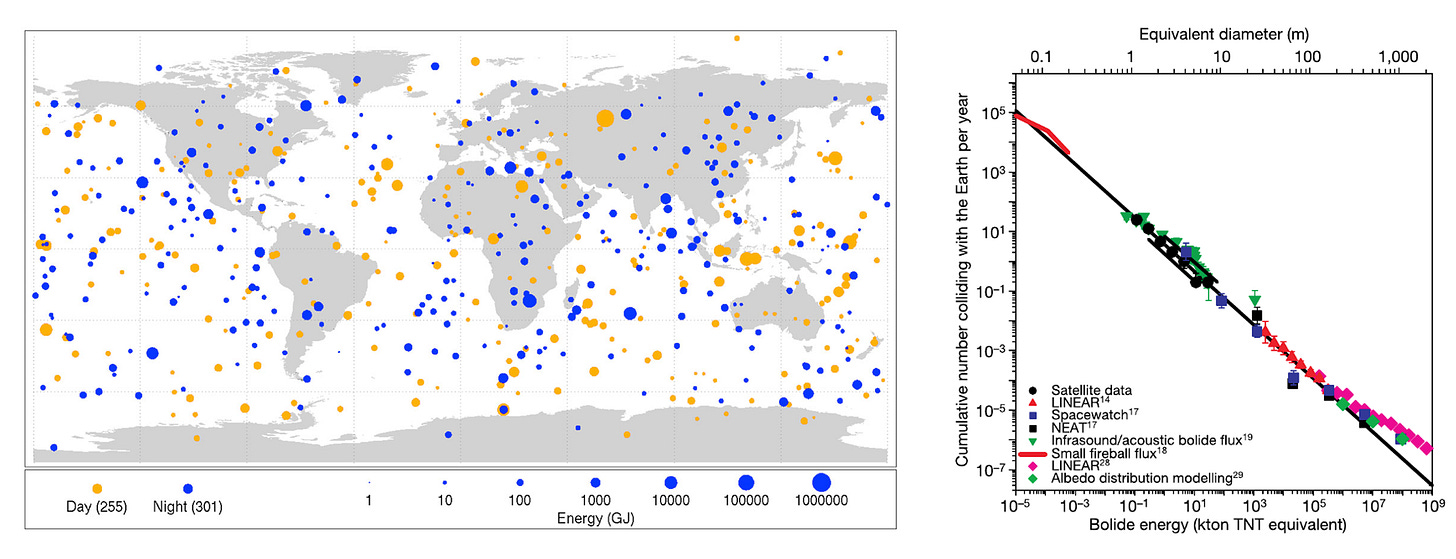
With AI, the unknowns relate to technological progress and governance rather than a physical system, so it isn’t clear how to model it mathematically. Still, people have tried. For example, in order to predict the computational requirements of a hypothetical AGI, severalworks assume that an AI system would require roughly as many computations as the human brain, and further make assumptions about the number of computations required by the human brain. These assumptions are far more tenuous than those involved in asteroid modeling, and none of this even addresses the loss-of-control question.
Without the reference classes or grounded theories, forecasts are necessarily “subjective probabilities”, that is, guesses based on the forecaster’s judgment. Unsurprisingly, these vary by orders of magnitude.
Subjective probability estimation does not get around the need for having either an inductive or a deductive basis for probability estimates. It merely avoids the need for the forecaster to explain their estimate. Explanation can be hard due to humans’ limited ability to explain our intuitive reasoning, whether inductive, deductive, or a combination thereof. Essentially, it allows the forecaster to say: “even though I haven’t shown my methods, you can trust this estimate because of my track record” (we explain in the next section why even this breaks down for AI x-risk forecasting). But ultimately, lacking either an inductive or a deductive basis, all that forecasters can do is to make up a number, and those made-up numbers are all over the place.
Consider the Existential Risk Persuasion Tournament (XPT) conducted by the Forecasting Research Institute in late 2022, which we think is the most elaborate and well-executed x-risk forecasting exercise conducted to date. It involved various groups of forecasters, including AI experts and forecasting experts (“superforecasters” in the figure). For AI experts, the high end (75th percentile) of estimates for AI extinction risk by 2100 is 12%, the median estimate is 3%, and the low end (25th percentile) is 0.25%. For forecasting experts, even the high end (75th percentile) is only 1%, the median is a mere 0.38%, and the low end (25th percentile) is visually indistinguishable from zero on the graph. In other words, the 75th percentile AI expert forecast and the 25th percentile superforecaster forecast differ by at least a factor of 100.
All of these estimates are from people who have deep expertise on the topic and participated in a months-long tournament where they tried to persuade each other! If this range of forecasts here isn’t extreme enough, keep in mind that this whole exercise was conducted by one group at one point in time. We might get different numbers if the tournament were repeated today, if the questions were framed differently, etc.
What’s most telling is to look at the rationales that forecasters provided, which are extensively detailed in the report. They aren’t using quantitative models, especially when thinking about the likelihood of bad outcomes conditional on developing powerful AI. For the most part, forecasters are engaging in the same kind of speculation that everyday people do when they discuss superintelligent AI. Maybe AI will take over critical systems through superhuman persuasion of system operators. Maybe AI will seek to lower global temperatures because it helps computers run faster, and accidentally wipe out humanity. Or maybe AI will seek resources in space rather than Earth, so we don’t need to be as worried. There’s nothing wrong with such speculation. But we should be clear that when it comes to AI x-risk, forecasters aren’t drawing on any special knowledge, evidence, or models that make their hunches more credible than yours or ours or anyone else’s.
The term superforecasting comes from Philip Tetlock’s 20 year study of forecasting (he was also one of the organizers of the XPT). Superforecasters tend to be trained in methods to improve forecasts such as by integrating diverse information and by minimizing psychological biases. These methods have been shown to be effective in domains such as geopolitics. But no amount of training will lead to good forecasts if there isn’t much useful evidence to draw from.
Even if forecasters had credible quantitative models (they don’t), they must account for “unknown unknowns”, that is, the possibility that the model itself might be wrong. As noted x-risk philosopher Nick Bostrom explains: “The uncertainty and error-proneness of our first-order assessments of risk is itself something we must factor into our all-things-considered probability assignments. This factor often dominates in low-probability, high-consequence risks — especially those involving poorly understood natural phenomena, complex social dynamics, or new technology, or that are difficult to assess for other reasons.”
This is a reasonable perspective, and AI x-risk forecasters do worry a lot about uncertainty in risk assessment. But one consequence of this is that for those who follow this principle, forecasts are guaranteed to be guesses rather than the output of a model — after all, no model can be used to estimate the probability that the model itself is wrong, or what the risk would be if the model were wrong.
To recap, subjective AI-risk forecasts vary by orders of magnitude. But if we can measure forecasters’ track records, maybe we can use that to figure out which forecasters to trust. In contrast to the previous two approaches for justifying risk estimates (inductive and deductive), the forecaster doesn’t have to explain their estimate, but instead justifies it based on their demonstrated skill at predicting other outcomes in the past.
This has proved to be invaluable in the domain of geopolitical events, and the forecasting community spends a lot of effort on skill measurement. Many ways to evaluate forecasting skill exist, such as calibration, the Brier score, the logarithmic score, or the Peer score used on the forecasting competition website Metaculus.
But regardless of which method is used, when it comes to existential risk, there are many barriers to assessing forecast skill for subjective probabilities: the lack of a reference class, the low base rate, and the long time horizon. Let’s look at each of these in turn.
Just as the reference class problem plagues the forecaster, it also affects the evaluator. Let’s return to the alien landing example. Consider a forecaster who has proved highly accurate at calling elections. Suppose this forecaster announces, without any evidence, that aliens will land on Earth within a year. Despite the forecaster’s demonstrated skill, this would not cause us to update our beliefs about an alien landing, because it is too dissimilar to election forecasting and we do not expect the forecaster’s skill to generalize. Similarly, AI x-risk is so dissimilar to any past events that have been forecast that there is no evidence of any forecaster’s skill at estimating AI x-risk.
Even if we somehow do away with the reference class problem, other problems remain — notably, the fact that extinction risks are “tail risks”, or risks that result from rare events. Suppose forecaster A says the probability of AI x-risk is 1%, and forecaster B says it is 1 in a million. Which forecast should we have more confidence in? We could look at their track records. Say we find that forecaster A (who has assigned a 1% probability to AI x-risk) has a better track record. It still doesn’t mean we should have more confidence in A’s forecast, because skill evaluations are insensitive to overestimation of tail risks. In other words, it could be that A scores higher overall because A is slightly better calibrated than B when it comes to everyday events that have a substantial probability of occurring, but tends to massively overestimate tail risks that occur rarely (for example, those with a probability of 1 in a million) by orders of magnitude. No scoring rule adequately penalizes this type of miscalibration.
Here’s a thought experiment to show why this is true. Suppose two forecasters F and G forecast two different sets of events, and the “true” probabilities of events in both sets are uniformly distributed between 0 and 1. We assume, highly optimistically, that both F and G know the true probability P[e] for every event e that they forecast. F always outputs P[e], but G is slightly conservative, never predicting a value less than 1%. That is, G outputs P[e] if P[e] >= 1%, otherwise outputs 1%.
By construction, F is the better forecaster. But would this be evident from their track records? In other words, many forecasts from each would we have to evaluate so that there’s a 95% chance that F outscores G? With the logarithmic scoring rule, it turns out to be on the order of a hundred million. With the Brier score, it is on the order of a trillion. We can quibble with the assumptions here but the point is that if a forecaster systematically overestimates tail risks, it is simply empirically undetectable.
The final barrier to assessing forecaster skill at predicting x-risk is that long-term forecasts take too long to evaluate (and extinction forecasts are of course impossible to evaluate). This can potentially be overcome. Researchers have developed a method called reciprocal scoring — where forecasters are rewarded based on how well they predict each others’ forecasts — and validate it in some real-world settings, such as predicting the effect of Covid-19 policies. In these settings, reciprocal scoring yielded forecasts that are as good as traditional scoring methods. Fair enough. But reciprocal scoring is not a way around the reference class problem or the tail risk problem.
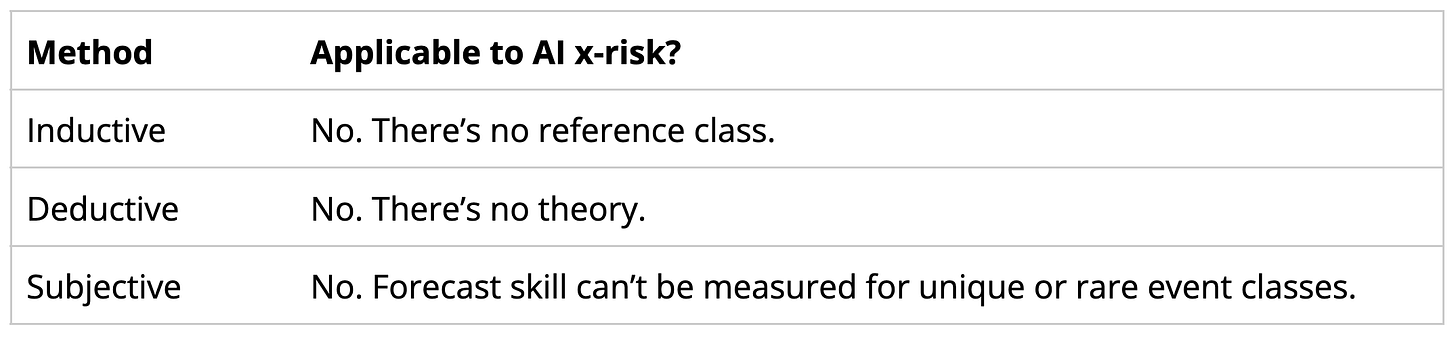
To recap, inductive and deductive methods don’t work, subjective forecasts are all over the place, and there’s no way to tell which forecasts are more trustworthy.
So in an attempt to derive more reliable estimates that could potentially inform policy, some researchers have turned to forecast aggregation methods that combine the predictions of multiple forecasters. A notable effort is the AI Impacts Survey on Progress in AI, but it has been criticized for serious methodological limitations including non-responsebias. More importantly, it is unclear why aggregation should improve forecast accuracy: after all, most forecasters might share the same biases (and again, none of them have any basis for a reliable forecast).
There are many reasons why forecasters might systematically overestimate AI x-risk. The first is selection bias. Take AI researchers: the belief that AI can change the world is one of the main motivations for becoming an AI researcher. And once someone enters this community, they are in an environment where that message is constantly reinforced. And if one believes that this technology is terrifyingly powerful, it is perfectly rational to think there is a serious chance that its world-altering effects will be negative rather than positive.
And in the AI safety subcommunity, which is a bit insular, the echo chamber can be deafening. Claiming to have a high p(doom) (one’s estimate of the probability of AI doom) seems to have become a way to signal one’s identity and commitment to the cause.
There is a slightly different selection bias at play when it comes to forecasting experts. The forecasting community has a strong overlap with effective altruism and concerns about existential risk, especially AI risk. This doesn’t mean that individual forecasters are biased. But having a high p(doom) might make someone more inclined to take up forecasting as an activity. So the community as a whole is likely biased toward people with x-risk worries.
Forecasters are good at updating their beliefs in response to evidence, but the problem is that unlike, say, asteroid impact risk, there is little evidence that can change one’s beliefs one way or another when it comes to AI x-risk, so we suspect that forecasts are strongly influenced by the priors with which people enter the community. The XPT report notes that “Few minds were changed during the XPT, even among the most active participants, and despite monetary incentives for persuading others.” In a follow-up study, they found that many of the disagreements were due to fundamental worldview differences that go beyond AI.
To reemphasize, our points about bias are specific to AI x-risk. If there were a community of election forecasters who were systematically biased (say, toward incumbents), this would become obvious after a few elections when comparing predictions with reality. But with AI x-risk, as we showed in the previous section, skill evaluation is insensitive to overestimation of tail risks.
Interestingly, skill evaluation is extremely sensitive to underestimation of tail risks: if you assign a probability of 0 for a rare event that actually ends up occurring, you incur an infinite penalty under the logarithmic scoring rule, from which you can never recover regardless of how well you predicted other events. This is considered one of the main benefits of the logarithmic score and is the reason it is adopted by Metaculus.
Now consider a forecaster who doesn’t have a precise estimate — and surely no forecaster has a precise estimate for something with so many axes of uncertainty as AI x-risk. Given the asymmetric penalties, the rational thing to do is to go with the higher end of their range of estimates.
In any case, it’s not clear what forecasters actually report when their estimates are highly uncertain. Maybe they don’t respond to the incentives of the scoring function. After all, long-term forecasts won’t be resolved anytime soon. And recall that in the case of the XPT, the incentive is actually to predict each others’ forecasts to get around the problem of long time horizons. The reciprocal scoring paper argues that this will incentivize forecasters to submit their true, high-effort estimates, and considers various objections to this claim. Their defense of the method rests on two key assumptions: that by exerting more effort forecasters can get closer to the true estimate, and that they have no better way to predict what other forecasters will do.
What if these assumptions are not satisfied? As we have argued throughout this post, with AI x-risk, we shouldn’t expect evidence to change forecasters’ prior beliefs, so the first assumption is dubious. And now that one iteration of the XPT has concluded, the published median estimates from that tournament serve as a powerful anchor (a “focal point” in game theory). It is possible that in the future, forecasters with reciprocal scoring incentives will use existing median forecasts as a starting point, only making minor adjustments to account for new information that has become available since the last tournament. The range of estimates might narrow as existing estimates serve as anchors for future estimates. All that is a roundabout way to say: the less actual evidence there is to draw upon, the more the risk of groupthink.
For what it’s worth, here are the median estimates from the XPT of both extinction risk and sub-extinction catastrophic risks from AI:
To reiterate, our view is that we shouldn’t take any of these numbers too seriously. They are a reflection of how much different samples of participants fret about AI than anything else.
As before, the estimates from forecasting experts (superforecasters) and AI experts differ by an order of magnitude or more. To the extent that we put any stock into these estimates, it should be the forecasting experts’ rather than the AI experts’ estimates. One important insight from past research is that domain experts perform worse than forecasting experts who have training in integrating diverse information and by minimizing psychological biases. Still, as we said above, even their forecasts may be vast overestimates, and we just can’t know for sure.
So what’s the big deal? So what if policymakers believe the risk over a certain timeframe is 1% instead of 0.01%? It seems pretty low in either case!
It depends on what they do with those probabilities. Most often, these estimates are merely a way to signal the fact that some group of experts thinks the risk is significant. If that’s all they are, so be it. But it’s not clear that all this elaborate effort at quantification is even helpful for this signaling purpose, given that different people interpret the same numbers wildly differently.
For example, Federal Trade Commission chair Lina Khan said her views on the matter were techno-optimistic since her p(doom) was only 15%, which left experts bewildered. (For what it’s worth, that number is about a thousandfold higher than what we would be comfortable labeling techno-optimist.) It takes a lot of quantitative training to be able to mentally process very small or very large numbers correctly in decision making, and not simply bucket them into categories like “insignificantly small”. Most people are not trained this way.
In short, what seems to be happening is that experts’ vague intuitions and fears are being translated into pseudo-precise numbers, and then translated back into vague intuitions and fears by policymakers. Let’s just cut the charade of quantification! The Center for AI Safety’s Statement on AI Risk was admirably blunt in this regard (of course, we strongly disagree with its substance).
A principled, quantitative way to use probabilities in decision making is utility maximization through cost-benefit analysis. The idea is simple: if we consider an outcome to have a subjective value, or utility, of U (which can be positive or negative), and it has, say, a 10% probability of occurring, we can act as if it is certain to occur and has a value of 0.1 * U. We can then add up the costs and benefits for each option available to us, and choose the one that maximizes costs minus benefits (the “expected utility”).
This is where things get really problematic. First, some people might consider extinction to have an unfathomably large negative value, because it precludes the existence of all the human lives, physical or simulated, that might ever be born in the future. The logical conclusion is that x-risk should be everyone’s top priority all the time! It is reminiscent of Pascal’s wager, the argument that it is rational believe in God because even if there is an infinitesimally small chance that God exists, the cost of non-belief is infinite (an eternity in hell as opposed to eternal happiness), and hence so is the expected utility. Fortunately, policymakers don’t give too much credence to decision making frameworks involving infinities. But the idea has taken a powerful hold of the AI safety community and drives some people’s conviction that AI x-risk should be society’s top priority.
Even if we limit ourselves to catastrophic but not existential risks, we are talking about billions of lives on the line, so the expected cost of even a 1% risk is so high that the policy implications are drastic — governments should increase spending on AI x-risk mitigation by orders of magnitude and consider draconian measures such as stopping AI development. This is why it is so vital to understand that these estimates are not backed by any methodology. It would be incredibly unwise to make world-changing policy decisions based on so little evidence.
Is there a role for forecasting in AI policy? We think yes — just not forecasting existential risk. Forecasting AI milestones, such as performance on certain capability benchmarks or economic impacts, is more achievable and meaningful. If a forecaster has demonstrated skill in predicting when various AI milestones would be reached, it does give us evidence that they will do well in the future. We are no longer talking about unique or rare events. And when considering lower-stakes policy interventions — preparing for potential economic disruption rather than staving off killer robots — it is less critical that forecasts be justified to the satisfaction of every reasonable person.
The forecasting community devotes a lot of energy to milestone forecasting. On Metaculus, the question “Will there be Human-machine intelligence parity before 2040?” has an aggregate prediction of 96% based on over 1,300 forecasters. That’s remarkable! If we agreed with this forecast, we would be in favor of the position that managing the safe transition to AGI should be a global priority. Why don’t we?
The answer is in the fine print. There is no consensus on the definition of a fuzzy concept such as AGI. Even if we fix a definition, determining whether it has been achieved can be hard or impossible. For effective forecasting, it is extremely important to avoid ambiguous outcomes. The way the forecasting community gets around this is by defining it in terms of relatively narrow skills, such as exam performance.
The Metaculus intelligence parity question is defined in terms of the performance on graduate exams in math, physics, and computer science. Based on this definition, we do agree with the forecast of 96%. But we think the definition is so watered down that it doesn’t mean much for policy. Forget existential risk — as we’ve written before, AI performance on exams has so little construct validity that it doesn’t even let us predict whether AI will replace workers.
Other benchmarks aren’t much better. In short, forecasting AI capability timelines is tricky because of the huge gap between benchmarks and real-world implications. Fortunately, better benchmarks reflecting consequential real-world tasks are being developed. In addition to benchmarks, we need naturalistic evaluation, even if it is more costly. One type of naturalistic evaluation is to measure how people perform their jobs differently with AI assistance. Directly forecasting economic, social, or political impacts — such as labor market transformation or AI-related spending by militaries — could be even more useful, although harder to unambiguously define and measure.
The responsibility for avoiding misuses of probability in policy lies with policymakers. We are not calling for forecasters to stop publishing forecasts in order to “protect” policymakers from being misled. That said, we think forecasts should be accompanied by a clear explanation of the process used and evidence considered. This would allow policymakers to make informed decisions about whether the justification presented meets the threshold that they are comfortable with. The XPT is a good example of transparency, as is this paper (though it is not about x-risk). On the other hand, simply surveying a bunch of researchers and presenting aggregate numbers is misinformative and should be ignored by policymakers.
So what should governments do about AI x-risk? Our view isn’t that they should do nothing. But they should reject the kind of policies that might seem compelling if we view x-risk as urgent and serious, notably: restricting AI development. As we’ll argue in a future essay in this series, not only are such policies unnecessary, they are likely to increase x-risk. Instead, governments should adopt policies that are compatible with a range of possible estimates of AI risk, and are on balance helpful even if the risk is negligible. Fortunately, such policies exist. Governments should also change policymaking processes so that they are more responsive to new evidence. More on all that soon.
The XPT report is titled Forecasting Existential Risks: Evidence from a Long-Run Forecasting Tournament.
Tetlock and Gardner’s book Superforecasting summarizes research by Tetlock, Barbara Mellers, and others.
Scott Alexander refutes the claim that there is something wrong in principle with ascribing probabilities to unique events (we largely agree). Our argument differs in two key ways from the position he addresses. We aren’t talking about forecasting in general; just its application to policymaking. And we don’t object to it in principle. Our argument is empirical: AI x-risk forecasts are extremely unreliable and lack justification. Theoretically this could change in the future, though we aren’t holding our breath.
A paper by Friedman and Zuckhauser explains why probabilities aren’t the whole story: two forecasts that have the same risk estimate might have very different implications for policymakers. In our view, AI x-risk forecasts fare poorly on two of the three dimensions of confidence: a sound basis in evidence and a narrow range of reasonable opinion.
A paper by our Princeton CITP colleagues led by Shazeda Ahmed explains the epistemic culture of AI safety, which consists of “cohesive, interwoven social structures of knowledge-production and community-building”. It helps understand why practices such as forecasting have become pillars of how the AI safety community forms its beliefs, as opposed to the broader scientific community that centers practices such as peer review. Of course, we shouldn’t reject a view just because it doesn’t conform to scientific orthodoxy. But at the same time, we shouldn’t give any deference to the self-styled AI safety community’s views on AI safety. It is important to understand that the median member of the AI safety community holds one particular stance on AI safety — a stance that is highly contested and in our view rather alarmist.
We have written extensively about evidence-based AI safety. Our best-known work includes the essay AI safety is not a model property and the paper titled On the Societal Impact of Open Foundation Models which was the result of a large collaboration.
Acknowledgements. We are grateful to Benjamin Edelman, Ezra Karger, Matt Salganik, and Ollie Stephenson for feedback on a draft. This series of essays is based on an upcoming paper that benefited from feedback from many people, including Seth Lazar and members of the MINT lab at Australian National University, students in the Limits to Prediction course at Princeton, and Shazeda Ahmed.
#2022, #Accidents, #ADD, #AGI, #Ai, #AIDevelopment, #AIModels, #AiSafety, #Alien, #Aliens, #Altruism, #Analysis, #Approach, #ArabSpring, #Assessment, #Asteroid, #Barrier, #Behavior, #Benchmarks, #Bias, #Book, #Born, #Brain, #Building, #Change, #Classes, #Collaboration, #Community, #Companies, #Comparison, #Competition, #Computer, #ComputerScience, #Computers, #Concrete, #Construction, #Course, #Covid, #Data, #Deal, #DecisionMaking, #Defense, #Democracy, #Details, #Development, #Dimensions, #Disruption, #Domains, #Dynamics, #Earth, #Echo, #Economic, #Effects, #Election, #Elections, #Energy, #Environment, #Events, #Exercise, #Explanation, #Extinction, #Factor, #Fair, #Federal, #Forecast, #Forms, #Foundation, #Fundamental, #Future, #Game, #GameTheory, #Gap, #Global, #Governance, #Government, #Graph, #Hand, #Horizon, #How, #HowTo, #Human, #HumanBehavior, #HumanBrain, #Humans, #Identity, #Impact, #Impacts, #IndustrialRevolution, #InSight, #Insights, #Insurance, #Intelligence, #It, #Jobs, #LaborMarket, #LED, #LESS, #London, #LowEnd, #Mass, #Math, #Matter, #Measure, #Members, #Message, #Method, #Milestone, #Milestones, #Mind, #Mitigation, #Model, #Modeling, #Models, #Natural, #Notes, #One, #OpenModels, #OPINION, #Other, #Paper, #Performance, #Persuasion, #Physics, #Play, #Policies, #Policy, #Power, #Precisely, #Predictions, #Probability, #Process, #Production, #Project, #Publishing, #Recover, #Reflection, #Report, #Research, #Resources, #Responsive, #Review, #Risk, #RiskAssessment, #Risks, #Robots, #Safety, #Science, #Scientific, #Searching, #Sensitive, #Signal, #Skills, #Social, #Society, #Sound, #Space, #Spectrum, #Spending, #Spring, #Students, #Study, #Sub, #Technology, #Temperatures, #Theory, #Time, #Trade, #Training, #Transformation, #Transition, #Transparency, #Trust, #University, #View, #Work, #X
Published on The Digital Insider at https://is.gd/FI2l1y.
Comments
Post a Comment
Comments are moderated.