Looking for a specific action in a video? This AI-based method can find it for you | By The Digital Insider
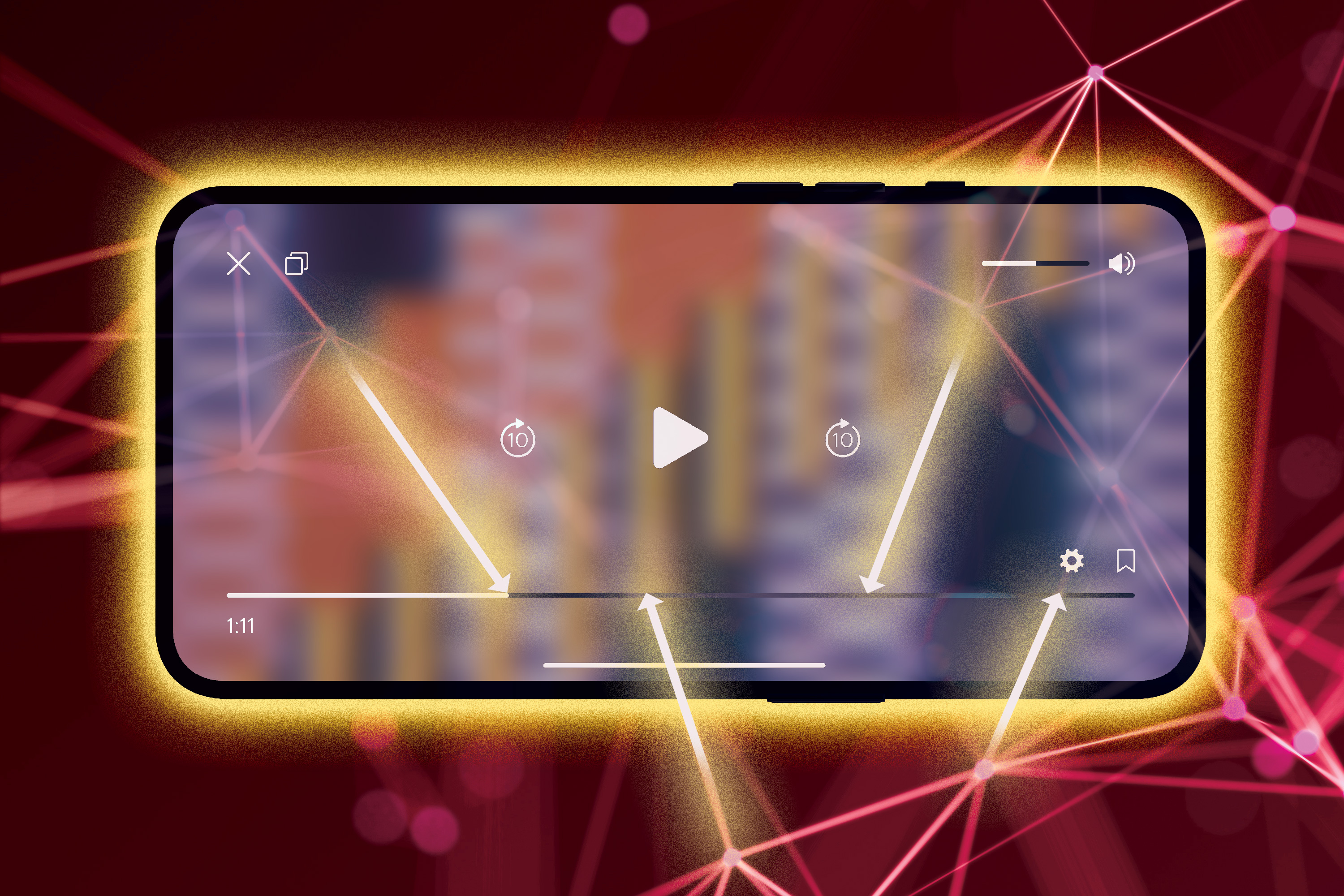
The internet is awash in instructional videos that can teach curious viewers everything from cooking the perfect pancake to performing a life-saving Heimlich maneuver.
But pinpointing when and where a particular action happens in a long video can be tedious. To streamline the process, scientists are trying to teach computers to perform this task. Ideally, a user could just describe the action they’re looking for, and an AI model would skip to its location in the video.
However, teaching machine-learning models to do this usually requires a great deal of expensive video data that have been painstakingly hand-labeled.
A new, more efficient approach from researchers at MIT and the MIT-IBM Watson AI Lab trains a model to perform this task, known as spatio-temporal grounding, using only videos and their automatically generated transcripts.
The researchers teach a model to understand an unlabeled video in two distinct ways: by looking at small details to figure out where objects are located (spatial information) and looking at the bigger picture to understand when the action occurs (temporal information).
Compared to other AI approaches, their method more accurately identifies actions in longer videos with multiple activities. Interestingly, they found that simultaneously training on spatial and temporal information makes a model better at identifying each individually.
In addition to streamlining online learning and virtual training processes, this technique could also be useful in health care settings by rapidly finding key moments in videos of diagnostic procedures, for example.
“We disentangle the challenge of trying to encode spatial and temporal information all at once and instead think about it like two experts working on their own, which turns out to be a more explicit way to encode the information. Our model, which combines these two separate branches, leads to the best performance,” says Brian Chen, lead author of a paper on this technique.
Chen, a 2023 graduate of Columbia University who conducted this research while a visiting student at the MIT-IBM Watson AI Lab, is joined on the paper by James Glass, senior research scientist, member of the MIT-IBM Watson AI Lab, and head of the Spoken Language Systems Group in the Computer Science and Artificial Intelligence Laboratory (CSAIL); Hilde Kuehne, a member of the MIT-IBM Watson AI Lab who is also affiliated with Goethe University Frankfurt; and others at MIT, Goethe University, the MIT-IBM Watson AI Lab, and Quality Match GmbH. The research will be presented at the Conference on Computer Vision and Pattern Recognition.
Global and local learning
Researchers usually teach models to perform spatio-temporal grounding using videos in which humans have annotated the start and end times of particular tasks.
Not only is generating these data expensive, but it can be difficult for humans to figure out exactly what to label. If the action is “cooking a pancake,” does that action start when the chef begins mixing the batter or when she pours it into the pan?
“This time, the task may be about cooking, but next time, it might be about fixing a car. There are so many different domains for people to annotate. But if we can learn everything without labels, it is a more general solution,” Chen says.
For their approach, the researchers use unlabeled instructional videos and accompanying text transcripts from a website like YouTube as training data. These don’t need any special preparation.
They split the training process into two pieces. For one, they teach a machine-learning model to look at the entire video to understand what actions happen at certain times. This high-level information is called a global representation.
For the second, they teach the model to focus on a specific region in parts of the video where action is happening. In a large kitchen, for instance, the model might only need to focus on the wooden spoon a chef is using to mix pancake batter, rather than the entire counter. This fine-grained information is called a local representation.
The researchers incorporate an additional component into their framework to mitigate misalignments that occur between narration and video. Perhaps the chef talks about cooking the pancake first and performs the action later.
To develop a more realistic solution, the researchers focused on uncut videos that are several minutes long. In contrast, most AI techniques train using few-second clips that someone trimmed to show only one action.
A new benchmark
But when they came to evaluate their approach, the researchers couldn’t find an effective benchmark for testing a model on these longer, uncut videos — so they created one.
To build their benchmark dataset, the researchers devised a new annotation technique that works well for identifying multistep actions. They had users mark the intersection of objects, like the point where a knife edge cuts a tomato, rather than drawing a box around important objects.
“This is more clearly defined and speeds up the annotation process, which reduces the human labor and cost,” Chen says.
Plus, having multiple people do point annotation on the same video can better capture actions that occur over time, like the flow of milk being poured. All annotators won’t mark the exact same point in the flow of liquid.
When they used this benchmark to test their approach, the researchers found that it was more accurate at pinpointing actions than other AI techniques.
Their method was also better at focusing on human-object interactions. For instance, if the action is “serving a pancake,” many other approaches might focus only on key objects, like a stack of pancakes sitting on a counter. Instead, their method focuses on the actual moment when the chef flips a pancake onto a plate.
Next, the researchers plan to enhance their approach so models can automatically detect when text and narration are not aligned, and switch focus from one modality to the other. They also want to extend their framework to audio data, since there are usually strong correlations between actions and the sounds objects make.
This research is funded, in part, by the MIT-IBM Watson AI Lab.
#2023, #Ai, #AiModel, #Approach, #Artificial, #ArtificialIntelligence, #Audio, #Benchmark, #Box, #Capture, #Challenge, #Computer, #ComputerScience, #ComputerScienceAndArtificialIntelligenceLaboratoryCSAIL, #ComputerScienceAndTechnology, #ComputerVision, #Computers, #Conference, #Cooking, #Data, #Deal, #Details, #Domains, #Edge, #ElectricalEngineeringComputerScienceEecs, #Focus, #Framework, #Glass, #Global, #Hand, #Health, #HealthCare, #Human, #Humans, #IBM, #IbmWatson, #Intelligence, #Internet, #It, #Kitchen, #Labels, #Language, #Learn, #Learning, #Life, #Liquid, #MachineLearning, #Method, #Mit, #MITSchwarzmanCollegeOfComputing, #MITIBMWatsonAILab, #Mitigate, #Mixing, #Model, #Moment, #Objects, #One, #OnlineLearning, #Other, #Paper, #Performance, #Picture, #Pieces, #Plan, #Process, #Research, #SchoolOfEngineering, #Science, #Sounds, #Stack, #Teaching, #Testing, #Text, #Time, #Tomato, #Training, #University, #Video, #Videos, #Vision, #Youtube
Published on The Digital Insider at https://is.gd/cuBhUI.
Comments
Post a Comment
Comments are moderated.