By Arvind Narayanan, Sayash Kapoor, and Rishi Bommasani.
The Biden-Harris administration has issued an executive order on artificial intelligence. It is about 20,000 words long and tries to address the entire range of AI benefits and risks. It is likely to shape every aspect of the future of AI, including openness: Will it remain possible to publicly release model weights while complying with the EO’s requirements? How will the EO affect the concentration of power and resources in AI? What about the culture of open research?
We cataloged the space of AI-related policies that might impact openness and grouped them into six categories. The EO includes provisions from all but one of these categories. Notably, it does not include licensing requirements. On balance, the EO seems to be good news for those who favor openness in AI.
But the devil is in the details. We will know more as agencies start implementing the EO. And of course, the EO is far from the only policy initiative worldwide that might affect AI openness.
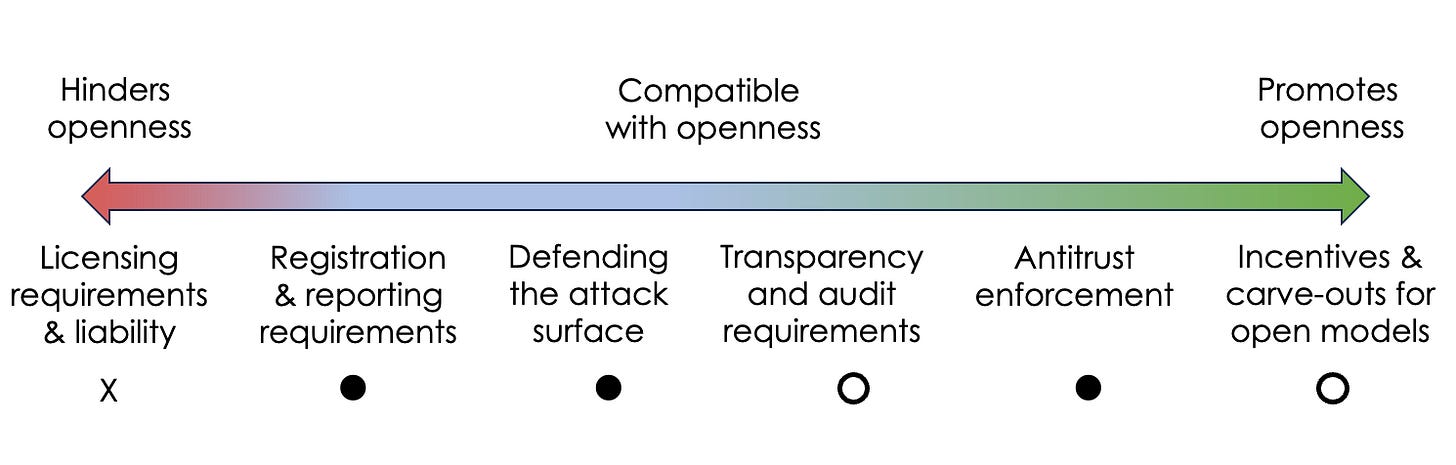
Licensing and liability
Licensing proposals aim to enable government oversight of AI by allowing only certain licensed companies and organizations to build and release state-of-the-art AI models. We are skeptical of licensing as a way of preventing the release of harmful AI: As the cost of training a model to a given capability level decreases, it will require increasingly draconian global surveillance to enforce.
Liability is closely related: The idea is that the government can try to prevent harmful uses by making model developers responsible for policing their use.
Both licensing and liability are inimical to openness. Sufficiently serious liability would amount to a ban on releasing model weights. Similarly, requirements to prevent certain downstream uses or to ensure that all generated content is watermarked would be impossible to satisfy if the weights are released.
Fortunately, the EO does not contain licensing or liability provisions. It doesn’t mention artificial general intelligence or existential risks, which have often been used as an argument for these strong forms of regulation.
The EO launches a public consultation process through the Department of Commerce to understand the benefits and risks of foundation models with publicly available weights. Based on this, the government will consider policy options specific to such models.
Registration and reporting
The EO does include a requirement to report to the government any AI training runs that are deemed large enough to pose a serious security risk. And developers must report various other details including the results of any safety evaluation (red-teaming) that they performed. Further, cloud providers need to inform the government when a foreign person attempts to purchase computational services that suffice to train a large enough model.
It remains to be seen how useful the registry will be for safety. It will depend in part on whether the compute threshold (any training run involving over 1026 mathematical operations is covered) serves as a good proxy for potential risk, and whether the threshold can be replaced with a more nuanced determination that evolves over time.
One obvious limitation is that once a model is openly released, fine tuning can be done far more cheaply, and can result in a model with very different behavior. Such models won’t need to be registered. There are many other potential ways for developers to architect around the reporting requirement if they chose to.
In general, we think it is unlikely that a compute threshold or any other predetermined criterion can effectively anticipate the riskiness of individual models. But in aggregate, the reporting requirement could give the government a better understanding of the landscape of risks.
The effects of the registry will also depend on how it is used. On the one hand it might be a stepping stone for licensing or liability requirements. But it might also be used for purposes more compatible with openness, which we discuss below.
The registry itself is not a deal breaker for open foundation models. All open models to date fall well below the compute threshold of 1026 operations. It remains to be seen if the threshold will stay frozen or change over time.
If the reporting requirements prove to be burdensome, developers will naturally try to avoid them. This might lead to a two-tier system for foundation models: frontier models whose size is unconstrained by regulation and sub-frontier models that try to stay just under the compute threshold to avoid reporting.
Defending attack surfaces
One possible defense against malicious uses of AI is to try to prevent bad actors from getting access to highly capable AI. We don’t think this will work. Another approach is to enumerate all the harmful ways in which such AI might be used, and to protect each target. We refer to this as defending attack surfaces. We have strongly advocated for this approach in our inputs to policy makers.
The EO has a strong and consistent emphasis on defense of attack surfaces, and applies it across the spectrum of risks identified: disinformation, cybersecurity, bio risk, financial risk, etc. To be clear, this is not the only defensive strategy that it adopts. There is also a strong focus on developing alignment methods to prevent models from being used for offensive purposes. Model alignment is helpful for closed models but less so for open models since bad actors can fine tune away the alignment.
Notable examples of defending attack surfaces:
The EO calls for methods to authenticate digital content produced by the federal government. This is a promising strategy. We think the big risk with AI-generated disinformation is not that people will fall for false claims — AI isn’t needed for that — but that people will stop trusting true information (the "liar's dividend"). Existing authentication and provenance efforts suffer from a chicken-and-egg problem, which the massive size of the federal government can help overcome.
It calls for the use of AI to help find and fix cybersecurity vulnerabilities in critical infrastructure and networks. Relatedly, the White House and DARPA recently launched a $20 million AI-for-cybersecurity challenge. This is spot on. Historically, the availability of automated vulnerability-discovery tools has helped defenders over attackers, because they can find and fix bugs in their software before shipping it. There’s no reason to think AI will be different. Much of the panic around AI has been based on the assumption that attackers will level-up using AI while defenders will stand still. The EO exposes the flaws of that way of thinking.
It calls for labs that sell synthetic DNA and RNA to better screen their customers. It is worth remembering that biological risks exist in the real world, and controlling the availability of materials may be far more feasible than controlling access to AI. These risks are already serious (for example, malicious actors already know how to create anthrax) and we already have ways to mitigate them, such as customer screening. We think it’s a fallacy to reframe existing risks (disinformation, critical infrastructure, bio risk) as AI risks. But if AI fears provide the impetus to strengthen existing defenses, that’s a win.
Transparency and audit
There is a glaring absence of transparency requirements in the EO — whether pre-training data, fine-tuning data, labor involved in annotation, model evaluation, usage, or downstream impacts. It only mentions red-teaming, which is a subset of model evaluation.
This is in contrast to another policy initiative also released yesterday, the G7 voluntary code of conduct for organizations developing advanced AI systems. That document has some emphasis on transparency.
Antitrust enforcement
The EO tasks federal agencies, in particular the Federal Trade Commission, with promoting competition in AI. The risks it lists include concentrated control of key inputs, unlawful collusion, and dominant firms disadvantaging competitors.
What specific aspects of the foundation model landscape might trigger these concerns remains to be seen. But it might include exclusive partnerships between AI companies and big tech companies; using AI functionality to reinforce walled gardens; and preventing competitors from using the output of a model to train their own. And if any AI developer starts to acquire a monopoly, that will trigger further concerns.
All this is good news for openness in the broader sense of diversifying the AI ecosystem and lowering barriers to entry.
Incentives for AI development
The EO asks the National Science Foundation to launch a pilot of the National AI Research Resource (NAIRR). The idea began as Stanford’s National Research Cloud proposal and has had a long journey to get to this point. NAIRR will foster openness by mitigating the resource gap between industry and academia in AI research.
Various other parts of the EO will have the effect of increasing funding for AI research and expanding the pool of AI researchers through immigration reform. (A downside of prioritizing AI-related research funding and immigration is increasing the existing imbalance among different academic disciplines. Another side effect is hastening the rebranding of everything as AI in order to qualify for special treatment, making the term AI even more meaningless.)
While we welcome the NAIRR and related parts of the EO, we should be clear that it falls far short of a full-throated commitment to keeping AI open. The North star would be a CERN style, well-funded effort to collaboratively develop open (and open-source) foundation models that can hold their own against the leading commercial models. Funding for such an initiative is probably a long shot today, but is perhaps worth striving towards.
What comes next?
We have described only a subset of the provisions in the EO, focusing on those that might impact openness in AI development. But it has a long list of focus areas including privacy and discrimination. This kind of whole-of-government effort is unprecedented in tech policy. It is a reminder of how much can be accomplished, in theory, with existing regulatory authority and without the need for new legislation.
The federal government is a distributed beast that does not turn on a dime. Agencies’ compliance with the EO remains to be seen. The timelines for implementation of the EO’s various provisions (generally 6-12 months) are simultaneously slow compared to the pace of change in AI, and rapid compared to the typical pace of policy making. In many cases it’s not clear if agencies have the funding and expertise to do what’s being asked of them. There is a real danger that it turns into a giant mess.
As a point of comparison, a 2020 EO required federal agencies to publish inventories of how they use AI — a far easier task compared to the present EO. Three years later, compliance is highly uneven and inadequate.
In short, the Biden-Harris EO is bold in its breadth and ambition, but it is a bit of an experiment, and we just have to wait and see what its effects will be.
Endnotes
We looked at the provisions for regulating AI discussed in each of the following papers and policy proposals, and clustered them into the six broad categories we discuss above:
Senators Blumenthal and Hawley's Bipartisan Framework for U.S. AI Act calls for licenses and liability as well as registration requirements for AI models.
A recent paper on the risks of open AI models by the Center for the Governance of AI advocates for licenses, liability, audits, and in some cases, asks developers not to release models at all.
A coalition of actors in the open-source AI ecosystem (Github, HF, EleutherAI, Creative Commons, LAION, and Open Future) put out a position paper responding to a draft version of the EU AI Act. The paper advocates for carve outs for open-source AI that exempt non-commercial and research applications of AI from liability, and it advocates for obligations (and liability) to fall on the downstream users of AI models.
In June 2023, the FTC shared its view on how it investigates antitrust and anti-competitive behaviors in the generative AI industry.
Transparency and auditing have been two of the main vectors for mitigating AI risks in the last few years.
Finally, we have previouslyadvocated for defending the attack surface to mitigate risks from AI.
Further reading
For a deeper look at the arguments in favor of registration and reporting requirements, see this paper or this short essay.
Widder, Whittaker, and West argue that openness alone is not enough to challenge the concentration of power in the AI industry.
#000, #2023, #Administration, #Ai, #AiTraining, #Antitrust, #Applications, #Approach, #Art, #Artificial, #ArtificialIntelligence, #Attackers, #Authentication, #Behavior, #Biden, #Bugs, #Challenge, #Chicken, #Cloud, #CloudProviders, #Code, #Commerce, #Companies, #Compliance, #Course, #CriticalInfrastructure, #Cybersecurity, #Data, #Deal, #Defense, #Details, #Developer, #Developers, #Development, #DigitalContent, #Disinformation, #DNA, #Effects, #Emphasis, #Eu, #ExecutiveOrder, #Financial, #FineTuning, #Forms, #Foundation, #Framework, #FTC, #Full, #Funding, #Future, #Gap, #Generative, #GenerativeAi, #Github, #Global, #Governance, #Government, #Hand, #How, #HowTo, #Immigration, #Impacts, #Industry, #Infrastructure, #Intelligence, #It, #Landscape, #LESS, #List, #Lists, #Materials, #Mathematical, #Model, #Networks, #News, #One, #OpenAi, #Organizations, #Other, #Panic, #Paper, #Pilot, #Policy, #Power, #Prevent, #Privacy, #Process, #Red, #Regulation, #Report, #Research, #Resources, #Risk, #Risks, #RNA, #Safety, #Science, #Security, #Software, #Space, #Spectrum, #Strategy, #Sub, #Subset, #Surface, #Surveillance, #Tech, #Theory, #Time, #Tools, #Trade, #Training, #Transparency, #Treatment, #Tuning, #Version, #View, #Vulnerabilities, #WhiteHouse, #Work
Published on The Digital Insider at https://bit.ly/3tObjms.
Comments
Post a Comment
Comments are moderated.