DeepSeek-V3: How a Chinese AI Startup Outpaces Tech Giants in Cost and Performance | By The Digital Insider
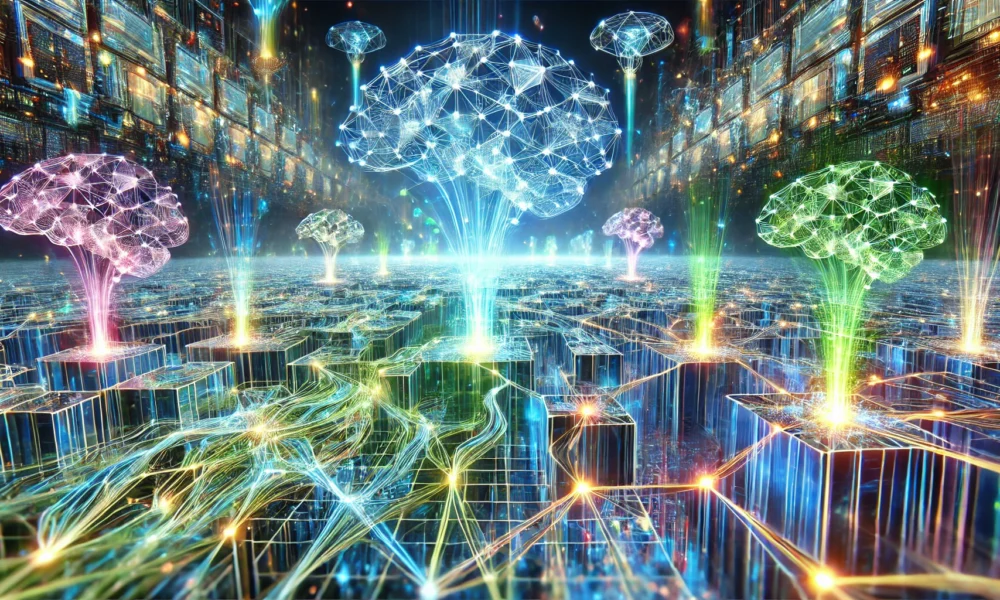
Generative AI is evolving rapidly, transforming industries and creating new opportunities daily. This wave of innovation has fueled intense competition among tech companies trying to become leaders in the field. US-based companies like OpenAI, Anthropic, and Meta have dominated the field for years. However, a new contender, the China-based startup DeepSeek, is rapidly gaining ground. With its latest model, DeepSeek-V3, the company is not only rivalling established tech giants like OpenAI’s GPT-4o, Anthropic’s Claude 3.5, and Meta’s Llama 3.1 in performance but also surpassing them in cost-efficiency. Besides its market edges, the company is disrupting the status quo by publicly making trained models and underlying tech accessible. Once secretly held by the companies, these strategies are now open to all. These developments are redefining the rules of the game.
In this article, we explore how DeepSeek-V3 achieves its breakthroughs and why it could shape the future of generative AI for businesses and innovators alike.
Limitations in Existing Large Language Models (LLMs)
As the demand for advanced large language models (LLMs) grows, so do the challenges associated with their deployment. Models like GPT-4o and Claude 3.5 demonstrate impressive capabilities but come with significant inefficiencies:
- Inefficient Resource Utilization:
Most models rely on adding layers and parameters to boost performance. While effective, this approach requires immense hardware resources, driving up costs and making scalability impractical for many organizations.
- Long-Sequence Processing Bottlenecks:
Existing LLMs utilize the transformer architecture as their foundational model design. Transformers struggle with memory requirements that grow exponentially as input sequences lengthen. This results in resource-intensive inference, limiting their effectiveness in tasks requiring long-context comprehension.
- Training Bottlenecks Due to Communication Overhead:
Large-scale model training often faces inefficiencies due to GPU communication overhead. Data transfer between nodes can lead to significant idle time, reducing the overall computation-to-communication ratio and inflating costs.
These challenges suggest that achieving improved performance often comes at the expense of efficiency, resource utilization, and cost. However, DeepSeek demonstrates that it is possible to enhance performance without sacrificing efficiency or resources. Here's how DeepSeek tackles these challenges to make it happen.
How DeepSeek-V3 Overcome These Challenges
DeepSeek-V3 addresses these limitations through innovative design and engineering choices, effectively handling this trade-off between efficiency, scalability, and high performance. Here’s how:
- Intelligent Resource Allocation Through Mixture-of-Experts (MoE)
Unlike traditional models, DeepSeek-V3 employs a Mixture-of-Experts (MoE) architecture that selectively activates 37 billion parameters per token. This approach ensures that computational resources are allocated strategically where needed, achieving high performance without the hardware demands of traditional models.
- Efficient Long-Sequence Handling with Multi-Head Latent Attention (MHLA)
Unlike traditional LLMs that depend on Transformer architectures which requires memory-intensive caches for storing raw key-value (KV), DeepSeek-V3 employs an innovative Multi-Head Latent Attention (MHLA) mechanism. MHLA transforms how KV caches are managed by compressing them into a dynamic latent space using “latent slots.” These slots serve as compact memory units, distilling only the most critical information while discarding unnecessary details. As the model processes new tokens, these slots dynamically update, maintaining context without inflating memory usage.
By reducing memory usage, MHLA makes DeepSeek-V3 faster and more efficient. It also helps the model stay focused on what matters, improving its ability to understand long texts without being overwhelmed by unnecessary details. This approach ensures better performance while using fewer resources.
- Mixed Precision Training with FP8
Traditional models often rely on high-precision formats like FP16 or FP32 to maintain accuracy, but this approach significantly increases memory usage and computational costs. DeepSeek-V3 takes a more innovative approach with its FP8 mixed precision framework, which uses 8-bit floating-point representations for specific computations. By intelligently adjusting precision to match the requirements of each task, DeepSeek-V3 reduces GPU memory usage and speeds up training, all without compromising numerical stability and performance.
- Solving Communication Overhead with DualPipe
To tackle the issue of communication overhead, DeepSeek-V3 employs an innovative DualPipe framework to overlap computation and communication between GPUs. This framework allows the model to perform both tasks simultaneously, reducing the idle periods when GPUs wait for data. Coupled with advanced cross-node communication kernels that optimize data transfer via high-speed technologies like InfiniBand and NVLink, this framework enables the model to achieve a consistent computation-to-communication ratio even as the model scales.
What Makes DeepSeek-V3 Unique?
DeepSeek-V3’s innovations deliver cutting-edge performance while maintaining a remarkably low computational and financial footprint.
- Training Efficiency and Cost-Effectiveness
One of DeepSeek-V3's most remarkable achievements is its cost-effective training process. The model was trained on an extensive dataset of 14.8 trillion high-quality tokens over approximately 2.788 million GPU hours on Nvidia H800 GPUs. This training process was completed at a total cost of around $5.57 million, a fraction of the expenses incurred by its counterparts. For instance, OpenAI's GPT-4o reportedly required over $100 million for training. This stark contrast underscores DeepSeek-V3's efficiency, achieving cutting-edge performance with significantly reduced computational resources and financial investment.
- Superior Reasoning Capabilities:
The MHLA mechanism equips DeepSeek-V3 with exceptional ability to process long sequences, allowing it to prioritize relevant information dynamically. This capability is particularly vital for understanding long contexts useful for tasks like multi-step reasoning. The model employs reinforcement learning to train MoE with smaller-scale models. This modular approach with MHLA mechanism enables the model to excel in reasoning tasks. Benchmarks consistently show that DeepSeek-V3 outperforms GPT-4o, Claude 3.5, and Llama 3.1 in multi-step problem-solving and contextual understanding.
- Energy Efficiency and Sustainability:
With FP8 precision and DualPipe parallelism, DeepSeek-V3 minimizes energy consumption while maintaining accuracy. These innovations reduce idle GPU time, reduce energy usage, and contribute to a more sustainable AI ecosystem.
Final Thoughts
DeepSeek-V3 exemplifies the power of innovation and strategic design in generative AI. By surpassing industry leaders in cost efficiency and reasoning capabilities, DeepSeek has proven that achieving groundbreaking advancements without excessive resource demands is possible.
DeepSeek-V3 offers a practical solution for organizations and developers that combines affordability with cutting-edge capabilities. Its emergence signifies that AI will not only be more powerful in the future but also more accessible and inclusive. As the industry continues to evolve, DeepSeek-V3 serves as a reminder that progress doesn’t have to come at the expense of efficiency.
#AffordableAI, #Ai, #AISustainability, #Anthropic, #Approach, #Architecture, #Article, #ArtificialIntelligence, #Attention, #Benchmarks, #Billion, #China, #Claude, #Claude3, #Claude35, #Communication, #Companies, #Competition, #Comprehension, #Computation, #ContextualUnderstanding, #CostEfficiency, #CostEfficientAI, #Cutting, #Data, #DataTransfer, #Deepseek, #DeepSeekV3, #Deployment, #Design, #Details, #Developers, #Developments, #Driving, #DualPipe, #Edge, #Efficiency, #Energy, #EnergyConsumption, #EnergyEfficiency, #Engineering, #Excel, #Financial, #FP8MixedPrecisionTraining, #FP8Precision, #Fraction, #Framework, #Future, #Game, #Generative, #GenerativeAi, #GPT, #Gpt4O, #Gpu, #GPUs, #Hardware, #How, #IdleTime, #Industries, #Industry, #Inference, #Innovation, #Innovations, #Investment, #It, #Language, #LanguageModels, #LargeLanguageModels, #LargeLanguageModelsLLMs, #Learning, #Limiting, #Llama, #Llama3, #Llama31, #LLMs, #Memory, #Meta, #MixedPrecisionTraining, #MixtureOfExperts, #Model, #ModelTraining, #Models, #Modular, #MoE, #MultiHeadLatentAttention, #Nvidia, #Openai, #Organizations, #Performance, #Power, #ProblemSolving, #Process, #ReinforcementLearning, #Resource, #ResourceAllocation, #Resources, #Rules, #Scale, #Space, #Speed, #Startup, #Sustainability, #Sustainable, #SustainableAI, #Tech, #Time, #Trade, #Training, #Transfer, #Transformer, #TransformerArchitecture, #TransformerArchitectures, #Transformers, #Units, #Wave
Published on The Digital Insider at https://is.gd/aAXv34.
Comments
Post a Comment
Comments are moderated.