Company leaders are eager to deploy generative AI (GenAI) in their businesses. So, why are so many projects failing to make it out of the proof of concept (POC) stage? At a recent Gartner event, Rita Sallam, distinguished vice-president analyst, said that at least 30% of GenAI projects will be dropped after POCs by the end of 2025 due to such issues as poor data quality, insufficient risk controls, fast-growing costs, or an inability to realize desired business value.
These issues are among the reasons why Gartner said GenAI is beginning to enter the trough of disillusionment in its latest Hype Cycle for Emerging Technology, 2024. However, in a separate Gartner survey, respondents reported that their GenAI deployments have helped companies notch 15.8% revenue increases, 15.2% cost savings, and 22.6% productivity improvements.
So, what separates enterprises that succeed in integrating GenAI into key workflows from those that fail to realize projected business value? These leaders and teams use a different approach characterized by rigorous preparation and change management. Here are three key principles to guide the evaluation, selection, and enablement of use cases with GenAI, so teams can mitigate risks and manage costs while transforming business processes.
1. Core principle 1: Rigorously quantify business value from the start:
While business leaders may have prioritized GenAI experimentation initially, they are now eager to reap tangible business value from investments.
Partners can help enterprises develop detailed business cases by holding workshops to understand overall goals, the current state of data processes and technology infrastructures, and more. As part of this process, they work with enterprise teams to evaluate potential use cases, prioritizing them by solving business pains, determining the level of effort and expected ROI, and developing key performance indicators to measure progress. At Google Cloud Next ’24, the company highlighted 101 stories of organizations succeeding with GenAI by deploying customer, employee, creative, data, code, and security agents.
Market capabilities continue to evolve, streamlining the path to value creation. Microsoft and Google have integrated large language models into their search engines. Internet users can now receive summarized answers and links, speeding their time to insight. Similarly, partners are offering GenAI accelerator platforms with AI and machine learning models that companies can customize and deploy in their environment within weeks. Enterprises benefit by gaining proven tools, reducing the cost and risk of deployment, and scaling new business capabilities faster.
2. Core principle 2: Ensure data quality, privacy, and security.
Providing high-quality, privacy-compliant, and secure data for model training and inference is the foundation of every successful GenAI implementation. Enterprises must prepare data to ensure AI models generate accurate and reliable outputs. In addition, they are implementing guardrails and new tools to protect sensitive information, including model outputs, from exposure. Similarly, GenAI can be used to identify security issues that can be remediated by teams or automation.
Mastercard is using GenAI to facilitate customer interactions and reduce fraud. Its AI-driven chatbots provide customers with instant access to personalized recommendations, account information, and transaction history.
The company also uses GenAI predictive modeling to identify unusual spending patterns, which could indicate potential fraud. With GenAI, Mastercard has doubled the detection rate of compromised cards; reduced false positives by up to 200%; and increased the speed of identifying merchants vulnerable to fraud by 300%.
3. Core principle 3: Strengthen human-GenAI collaboration.
While GenAI will automate some processes, most of the time, it will assist humans in making better decisions. GenAI can create synthetic data, process data, recognize patterns, and create predictive analytics to empower teamwork and the creation of new services. For example, GenAI can provide scenarios and recommendations for decision-makers to consider so that they can optimize outcomes. Humans bring marketplace and contextual awareness, business knowledge, judgment, and empathy to decision-making, building on GenAI capabilities.
So, how can companies maximize the potential of human-GenAI collaborations? Leaders should take the time to set up clearly defined roles and responsibilities, continuously train teams on the latest capabilities, and provide guardrails and escalation paths when GenAI doesn’t perform as expected. In addition, they should share their vision for GenAI reshaping the business and stress that they are augmenting human capabilities rather than replacing them. A Forrester survey found that 36% of employees fear losing their jobs to automation or AI, but only 1.5% will, while 6.5% will have their roles influenced by GenAI. As a result, employees should embrace this technology rather than shun it.
Allstate has implemented a GenAI-powered chatbot that leverages natural language processing to deliver real-time, multilingual support and gain greater insight into customer behavior. For example, it seeks to improve the performance of previous models threefold by identifying those customer journeys that require agent support.
The chatbot streamlines the claims process by providing a centralized platform for gathering and reviewing relevant information. While human agents continue to handle complex claims requiring expert judgment, the chatbot significantly enhances efficiency by automating routine tasks and reducing processing time. By using AI to streamline form completion, Allstate is improving accuracy and customer satisfaction.
Reap More ROI from GenAI by Adopting These 3 Core Principles
When GenAI burst into the world’s consciousness, leaders quickly applied it to these businesses, encouraging experimentation and innovation. However, sometimes POCs raced ahead of fundamentals, escalating costs and creating solutions that didn’t deliver the desired value.
Leaders can use these three core principles – developing a sound business case, addressing data requirements, and helping teams collaborate with AI – to make new GenAI initiatives successful. They’ll be able to point to high-value use cases and tools, data safeguards, and productivity and innovation improvements that thrill the C-suite, boards, customers, and investors alike.
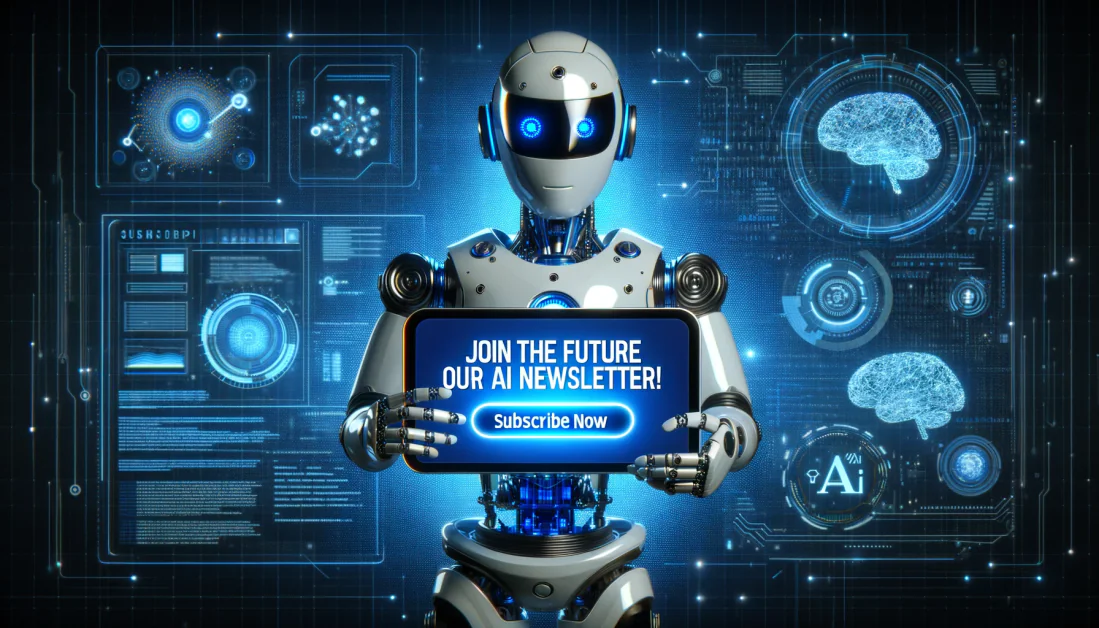
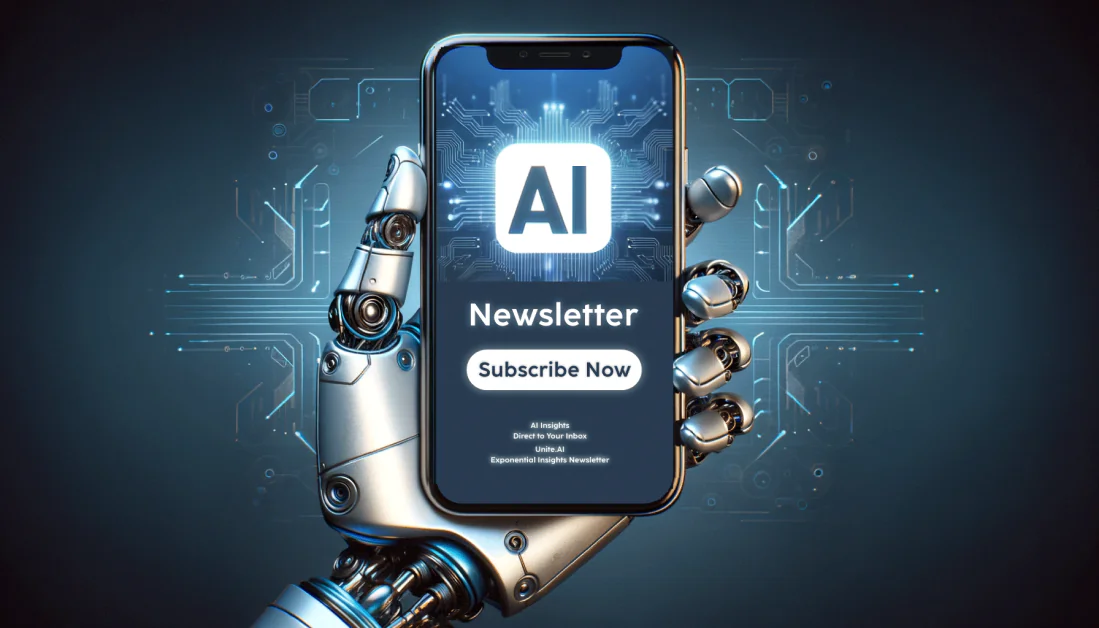
#2024, #Agent, #Agents, #Ai, #AIModels, #Analytics, #Approach, #Automation, #Awareness, #Behavior, #Boards, #Building, #Business, #CSuite, #Change, #ChangeManagement, #Chatbot, #Chatbots, #Cloud, #Code, #Collaborate, #Collaboration, #Companies, #Consciousness, #CostSavings, #Data, #DataQuality, #DecisionMakers, #Deploying, #Deployment, #Detection, #Efficiency, #EmergingTechnology, #Empathy, #Employees, #Engines, #Enterprise, #Enterprises, #Environment, #Evaluation, #Event, #FalsePositives, #Fear, #Form, #Forrester, #Foundation, #Fraud, #Gartner, #Genai, #Generative, #GenerativeAi, #Google, #GoogleCloud, #GoogleCloudNext, #History, #How, #Human, #Humans, #Inference, #Innovation, #InSight, #Internet, #Investments, #Issues, #It, #Jobs, #Language, #LanguageModels, #LargeLanguageModels, #LatentView, #LatentViewAnalytics, #Learning, #Links, #MachineLearning, #Management, #Measure, #Microsoft, #Mitigate, #Model, #ModelTraining, #Modeling, #Models, #Natural, #NaturalLanguage, #NaturalLanguageProcessing, #Organizations, #Partners, #Patterns, #Performance, #Platform, #PoC, #PoorDataQuality, #PredictiveAnalytics, #PredictiveModeling, #President, #Privacy, #Process, #Productivity, #ProofOfConcept, #RealTime, #Revenue, #Risk, #Risks, #ROI, #Roles, #Savings, #Scaling, #Search, #SearchEngines, #Security, #Sensitive, #SensitiveInformation, #Sound, #Speed, #Spending, #Stories, #Stress, #SyntheticData, #Teams, #Teamwork, #Technology, #ThoughtLeaders, #Time, #Tools, #Training, #Transaction, #Vision, #Work, #Workflows, #Workshops, #World
Published on The Digital Insider at https://is.gd/iLwCeW.
Comments
Post a Comment
Comments are moderated.